Latest Advancements in Image-to-Video Conversion
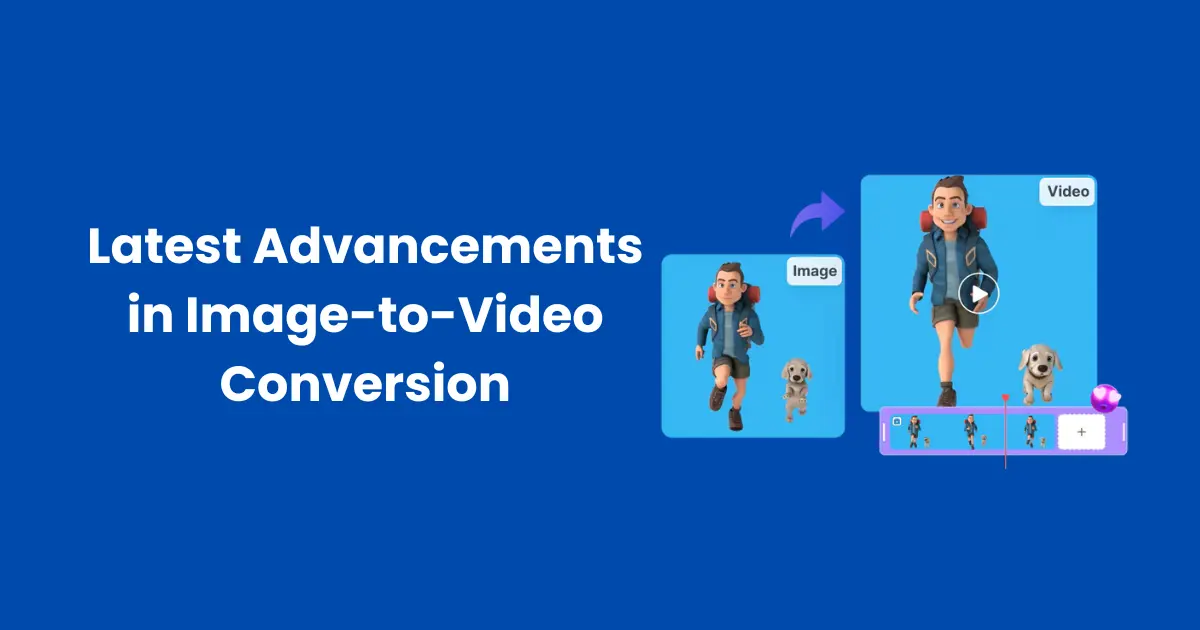
Contents
- 1 How Image-to-Video Conversion Works
- 2 Latest Advancements in Image-to-Video Conversion
- 3 Top Tools and Software for Image-to-Video Conversion
- 4 Applications of Image-to-Video Conversion
- 5 Challenges in Image-to-Video Conversion
- 6 Future Trends in Image-to-Video Conversion
- 6.1 1. AI-Driven Motion Prediction and Enhancement
- 6.2 2. Real-Time Image-to-Video Generation
- 6.3 3. Integration with Text-to-Video Technology
- 6.4 4. Hyper-Realistic Facial Animation for Digital Avatars
- 6.5 5. Enhanced 3D and Depth Perception
- 6.6 6. Ethical AI and Deepfake Detection
- 6.7 7. Democratization of AI Video Creation
- 6.8 Conclusion
The latest advancements in image-to-video conversion have revolutionized the way static visuals are transformed into dynamic sequences. With the rapid progress of artificial intelligence (AI) and machine learning, generating high-quality videos from images has become more efficient and realistic. As a result, industries such as animation, marketing, and content creation are experiencing significant improvements in workflow and creativity.
In recent years, image-to-video conversion has benefited from deep learning algorithms, neural networks, and motion prediction techniques. These advancements enable smoother transitions, better frame interpolation, and enhanced realism in generated videos. As AI-driven models continue to evolve, users can now create high-resolution, lifelike animations from a single image with minimal effort.
This article explores the latest advancements in image-to-video conversion, discussing the technologies behind them, the top tools available, real-world applications, and future trends. Let’s dive into how this groundbreaking technology is reshaping digital content creation.
How Image-to-Video Conversion Works
The process of image-to-video conversion relies on advanced artificial intelligence, deep learning models, and motion prediction techniques to generate smooth video sequences from static images. These technologies analyze image features, predict motion, and interpolate frames to create realistic animations.

Key Technologies Behind the Process
- Neural Networks and Deep Learning
- AI-powered neural networks analyze the input image, detect objects, and estimate motion paths.
- Deep learning models, such as Generative Adversarial Networks (GANs) and Convolutional Neural Networks (CNNs), generate realistic movements.
- Frame Interpolation Techniques
- AI estimates missing frames by predicting intermediate transitions, ensuring smooth animation.
- Advanced interpolation methods like Depth-Aware Video Frame Interpolation (DAIN) enhance motion fluidity.
- Optical Flow Algorithms
- These algorithms analyze pixel movement between frames to generate realistic motion sequences.
- Optical flow is crucial for maintaining temporal consistency in AI-generated videos.
- Motion Estimation and Prediction
- AI models predict how objects in the image would move naturally based on training data.
- Realistic movements are achieved using physics-based simulations and facial motion tracking.
With these cutting-edge technologies, image-to-video conversion enables the creation of lifelike animations, making it a valuable tool in digital content production. The next section explores the latest advancements in image-to-video conversion that are shaping the future of video generation.
Latest Advancements in Image-to-Video Conversion
The field of image-to-video conversion has seen remarkable improvements due to breakthroughs in artificial intelligence, deep learning, and computer vision. These advancements have made it possible to generate highly realistic, smooth, and dynamic video content from static images. Below are some of the most significant developments in this field.
1. AI-Powered Motion Prediction
Recent AI models can accurately predict motion based on an image’s content. By analyzing facial expressions, body postures, and scene elements, deep learning algorithms generate natural movements. Transformers and recurrent neural networks (RNNs) are now used to create more precise motion sequences, significantly enhancing the realism of AI-generated videos.
2. Realistic Frame Interpolation Techniques
Frame interpolation plays a crucial role in image-to-video conversion. Modern AI-based interpolation techniques, such as Depth-Aware Video Frame Interpolation (DAIN) and RIFE (Real-Time Intermediate Flow Estimation), produce ultra-smooth animations by filling in missing frames with near-perfect accuracy. These techniques improve motion flow, reducing artifacts and unnatural distortions.
3. Text-to-Animation Integrations
A revolutionary advancement is the integration of text-based commands into image-to-video conversion. AI models like Runway ML and Stable Video Diffusion allow users to input textual prompts to guide motion, camera angles, and scene dynamics. This development simplifies animation creation, making it accessible to content creators with minimal expertise.
4. Enhancements in Temporal Consistency
Earlier AI-generated videos often suffered from inconsistencies in motion, leading to flickering or unnatural transitions. New models now prioritize temporal coherence, ensuring that each frame smoothly connects to the next. Techniques such as latent diffusion models (LDMs) and flow-based motion smoothing have significantly improved this aspect of image-to-video conversion.
5. AI-Driven 3D Animation from 2D Images
Recent research has enabled the conversion of single 2D images into 3D animated videos. AI models like NVIDIA’s Instant NeRF (Neural Radiance Fields) reconstruct three-dimensional depth and create 360-degree animations from a static image. This advancement is particularly useful for game development, AR/VR applications, and photorealistic content generation.
These latest advancements in image-to-video conversion are transforming digital content creation, making video production faster, more efficient, and highly accessible. In the next section, we will explore some of the top tools and software that leverage these cutting-edge technologies.
Top Tools and Software for Image-to-Video Conversion
The latest advancements in image-to-video conversion have led to the development of powerful tools that leverage AI and deep learning to create high-quality animated videos. These tools enable users to transform static images into dynamic sequences with minimal effort. Below are some of the most innovative and widely used software solutions in this field.

1. Runway ML
- Overview: A cutting-edge AI-powered tool that simplifies video generation and motion creation.
- Key Features:
- AI-assisted image animation and motion effects.
- Text-to-video capabilities for customized animation.
- Cloud-based platform with an intuitive interface.
- Best For: Content creators, marketers, and designers looking for AI-driven video generation.
2. Deep Nostalgia (MyHeritage AI)
- Overview: Uses AI to animate old photographs by generating natural facial expressions and movements.
- Key Features:
- AI-powered facial motion synthesis.
- Automatic animation of historical images.
- Smooth and realistic motion effects.
- Best For: Bringing old family photos to life with subtle yet realistic animations.
3. DAIN (Depth-Aware Video Frame Interpolation)
- Overview: A deep learning-based tool that enhances motion fluidity by generating intermediate frames.
- Key Features:
- AI-driven frame interpolation for smoother animations.
- Advanced depth-aware technology.
- Supports high-resolution video generation.
- Best For: Enhancing image-to-video conversions with high-quality motion effects.
4. Adobe After Effects (AI Plugins)
- Overview: A professional video editing and animation tool with AI-powered plugins.
- Key Features:
- AI-based motion tracking and interpolation.
- Integration with third-party AI animation tools.
- High customization options for advanced video production.
- Best For: Professional animators and filmmakers seeking precise control over AI-assisted video creation.
5. Pika Labs
- Overview: A recent AI-powered tool that generates short animations from images.
- Key Features:
- AI-assisted motion effects for still images.
- Simple interface with fast processing.
- Works well for social media content and artistic animations.
- Best For: Quick and creative animation effects for short-form content.
These top tools for image-to-video conversion are transforming video production by making AI-assisted animation accessible to professionals and beginners alike. In the next section, we will explore real-world applications of image-to-video conversion across different industries.
Applications of Image-to-Video Conversion
The latest advancements in image-to-video conversion have significantly impacted various industries, making content creation more efficient and dynamic. AI-powered tools are being integrated into multiple fields, from entertainment to historical preservation. Below are some of the most prominent applications of image-to-video conversion.

1. Animation and Film Industry
- AI-generated videos reduce the time and effort required for traditional animation.
- Image-to-video tools assist in character animation, background motion, and special effects.
- AI-powered motion prediction enhances frame interpolation for smoother transitions.
2. Marketing and Advertising
- Brands use image-to-video conversion to create engaging social media content from static images.
- AI animation tools enable the rapid production of promotional videos, increasing audience engagement.
- Businesses can personalize video ads by animating customer-related visuals.
3. Social Media Content Creation
- Influencers and digital marketers use AI tools to animate images for engaging video posts.
- Platforms like TikTok and Instagram benefit from quick, AI-generated video effects.
- Image-to-video technology enhances storytelling by bringing still images to life.
4. Historical Image Revitalization
- AI-driven video conversion is used to animate historical photos, making history more immersive.
- Museums and documentary creators use motion synthesis to reconstruct past events visually.
- Deep Nostalgia and similar tools bring old photographs to life with realistic facial animations.
5. Gaming and Virtual Reality (VR)
- Game developers use AI to animate characters and environments from concept art.
- AI-driven video conversion enables real-time animation in VR experiences.
- Motion prediction enhances character interactions in immersive digital worlds.
6. Medical and Scientific Visualization
- AI-powered image-to-video conversion helps in medical imaging, simulating body movements from CT scans and X-rays.
- Researchers use AI-generated videos for scientific animations to visualize complex data.
- Training programs benefit from animated simulations of biological processes.
The integration of image-to-video conversion across these industries is reshaping content creation, making video production faster and more accessible. In the next section, we will explore some of the key challenges faced in image-to-video conversion and how AI is working to overcome them.
Challenges in Image-to-Video Conversion
Despite the latest advancements in image-to-video conversion, the technology still faces several challenges that impact its accuracy, realism, and efficiency. These challenges stem from AI limitations, data quality issues, and computational demands. Below are the key obstacles and how researchers are working to overcome them.
1. Maintaining Temporal Consistency
- One of the biggest challenges in image-to-video conversion is ensuring that animations remain consistent across frames.
- AI-generated videos sometimes suffer from flickering, unnatural transitions, or sudden motion distortions.
- Solution: Advanced deep learning techniques, such as latent diffusion models (LDMs) and flow-based motion smoothing, help improve temporal coherence.
2. Realistic Motion Generation
- Predicting natural movement from a static image is complex, especially for human animations.
- AI models often struggle with generating realistic body motion, facial expressions, and object interactions.
- Solution: New models, such as transformers and neural physics engines, are improving motion realism by learning from vast datasets of real-world movements.
3. High Computational Requirements
- Image-to-video conversion requires powerful GPUs and high-end computing resources.
- AI-based tools demand extensive processing power, making real-time applications challenging.
- Solution: Cloud-based platforms like Runway ML and Google’s AI research models provide scalable processing, reducing the burden on local hardware.
4. Handling Complex Backgrounds and Depth Perception
- AI often struggles with depth estimation, leading to unnatural animations when handling complex backgrounds.
- Converting 2D images into 3D-like video sequences remains a technical challenge.
- Solution: Neural Radiance Fields (NeRFs) and multi-frame depth estimation models are improving the ability to simulate depth accurately.
5. Ethical and Misinformation Concerns
- AI-powered image-to-video conversion can be misused to create deepfakes, raising ethical concerns.
- Misinformation through manipulated videos is a growing issue in media and politics.
- Solution: Researchers are developing AI-based detection tools to identify fake videos and prevent unethical use.
While these challenges persist, rapid advancements in AI, deep learning, and computer vision continue to refine image-to-video conversion, making it more reliable and accessible. In the next section, we will explore the future trends and possibilities shaping this technology.
Future Trends in Image-to-Video Conversion
As technology evolves, the latest advancements in image-to-video conversion are expected to bring revolutionary changes across various industries. AI-driven innovations will enhance realism, reduce processing time, and expand creative possibilities. Below are the key trends shaping the future of image-to-video conversion.

1. AI-Driven Motion Prediction and Enhancement
- Future AI models will improve motion synthesis, making animations more fluid and natural.
- Neural physics engines will help predict human movement with greater accuracy.
- Enhanced GANs (Generative Adversarial Networks) will refine frame transitions, eliminating motion distortions.
2. Real-Time Image-to-Video Generation
- Current AI models require significant processing time, but future advancements will enable instant image-to-video conversion.
- Edge AI computing will allow devices to generate videos locally without relying on cloud-based systems.
- This will benefit industries like gaming, augmented reality (AR), and virtual reality (VR).
3. Integration with Text-to-Video Technology
- AI-powered image-to-video conversion will merge with text-to-video tools, allowing users to generate dynamic animations from textual descriptions and static images.
- Platforms like Runway ML and Pika Labs are already exploring this possibility.
- This trend will enable automated storytelling, personalized marketing, and AI-generated filmmaking.
4. Hyper-Realistic Facial Animation for Digital Avatars
- AI will create ultra-realistic avatars by enhancing facial animations derived from static images.
- Technologies like Deep Neural Rendering will make digital characters almost indistinguishable from real humans.
- This will impact industries such as gaming, virtual influencers, and metaverse applications.
5. Enhanced 3D and Depth Perception
- AI will improve 2D-to-3D video conversion, allowing for depth-aware video generation from single images.
- Neural Radiance Fields (NeRFs) will enable AI models to reconstruct complex 3D environments with greater accuracy.
- This advancement will be particularly useful in VR, animation, and digital cinematography.
6. Ethical AI and Deepfake Detection
- With the rise of image-to-video conversion, ethical concerns regarding deepfakes and misinformation will increase.
- Future AI models will integrate watermarking and verification tools to distinguish real from AI-generated content.
- AI-driven regulatory frameworks will emerge to prevent the misuse of video generation technology.
7. Democratization of AI Video Creation
- AI-powered image-to-video conversion tools will become more accessible to everyday users.
- No-code and low-code AI platforms will allow non-experts to create professional-quality animations effortlessly.
- This trend will revolutionize content creation, e-learning, and online marketing.
The future of image-to-video conversion is filled with exciting possibilities, driven by AI advancements in motion prediction, real-time processing, and deepfake detection. As these technologies evolve, they will redefine how we create, consume, and interact with video content. Embracing these trends will open new creative opportunities across multiple industries.
Conclusion
The latest advancements in image-to-video conversion are revolutionizing content creation by making video production more accessible, efficient, and realistic. AI-driven innovations have enhanced motion prediction, real-time processing, and depth perception, allowing for more seamless and high-quality video generation.
Despite these breakthroughs, challenges such as temporal consistency, high computational demands, and ethical concerns remain. However, continuous improvements in deep learning models, GANs, and neural rendering are addressing these issues, making image-to-video conversion more reliable and widely applicable.
As AI continues to evolve, future trends indicate the rise of real-time video generation, hyper-realistic animations, and AI-powered storytelling. These advancements will reshape industries like entertainment, marketing, education, and virtual reality, offering new creative possibilities.
Embracing these innovations will not only enhance digital experiences but also pave the way for a future where image-to-video conversion becomes an integral part of visual storytelling and media production.