Image-to-Video Synthesis Techniques: A Comprehensive Guide
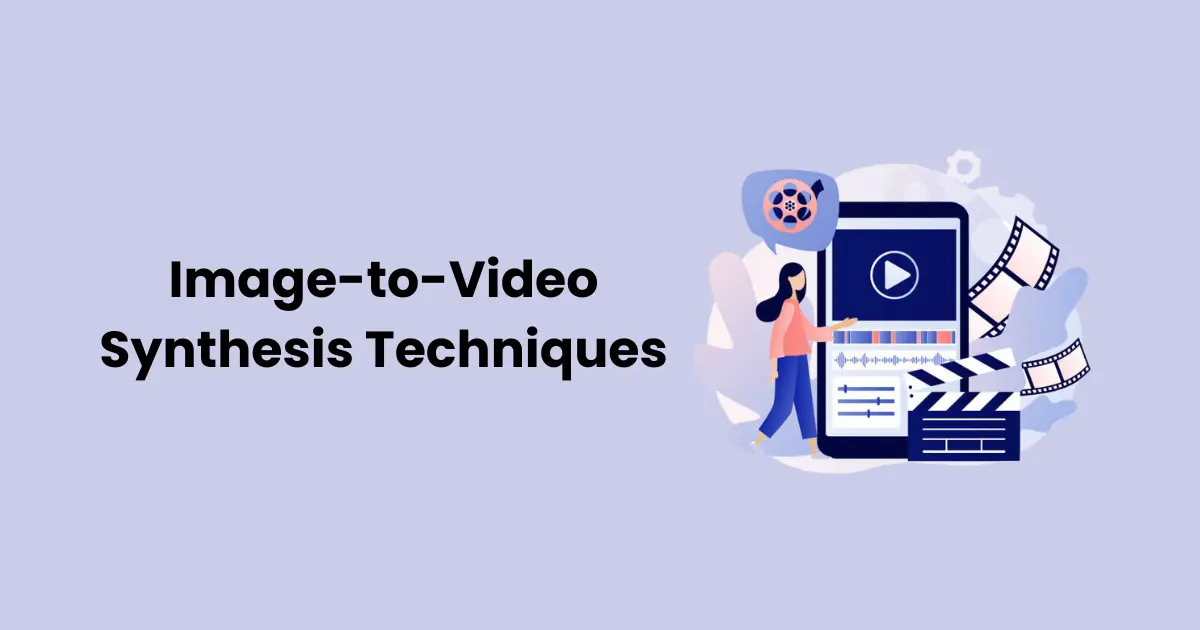
Contents
The rise of artificial intelligence has transformed digital content creation, and one of the most fascinating advancements is image-to-video synthesis techniques. These methods enable the transformation of static images into dynamic videos by applying artificial motion, interpolation, and deep learning algorithms. With advancements in AI, image-to-video synthesis techniques have become increasingly sophisticated, allowing for the generation of realistic and high-quality animations.
From enhancing visual storytelling to automating content creation, these techniques are revolutionizing industries such as entertainment, marketing, and gaming. By leveraging neural networks and motion prediction models, image-to-video synthesis techniques can bring still images to life with fluid movement. In this article, we will explore how these methods work, the most common approaches, their real-world applications, and what the future holds for this rapidly evolving technology.
What Is Image-to-Video Synthesis?
Image-to-video synthesis is the process of generating a video sequence from a single static image or a set of images. This technology utilizes artificial intelligence (AI), deep learning, and motion prediction algorithms to create smooth and realistic motion from still visuals. By analyzing the features of an image, image-to-video synthesis techniques predict movement patterns and generate frames that simulate natural transitions, making the static image appear dynamic.
This process is widely used in various applications, including animation, video editing, and virtual reality. AI-powered models, such as Generative Adversarial Networks (GANs) and neural rendering, help enhance realism by predicting object movement, adjusting lighting effects, and synthesizing new frames. The ability to transform a simple image into a dynamic video opens up new possibilities in creative content generation, automation, and immersive media experiences.
How Image-to-Video Synthesis Works
Key Steps in Image-to-Video Generation
Image-to-video synthesis techniques rely on multiple computational processes to create realistic motion from still images. The workflow typically involves the following key steps:

- Image Analysis – AI models analyze the input image to identify key features, such as edges, textures, objects, and depth information.
- Motion Estimation – Using deep learning algorithms, the system predicts how different parts of the image should move over time. This may involve estimating trajectories, deformations, or simulating natural physics.
- Frame Generation – The model synthesizes intermediate frames by interpolating motion between the original image and predicted positions.
- Temporal Smoothing – To ensure fluid motion, AI algorithms refine transitions between frames, removing jitter or unnatural distortions.
- Rendering and Output – Once the sequence is complete, post-processing techniques enhance visual quality, adjusting lighting, texture consistency, and frame rate.
Role of AI and Deep Learning
AI-driven image-to-video synthesis techniques heavily depend on machine learning and deep learning approaches, such as:
- Generative Adversarial Networks (GANs): These models generate realistic motion by training on vast datasets of video sequences. They predict plausible movement patterns and create new frames accordingly.
- Recurrent Neural Networks (RNNs) & Transformers: These models help in time-sequence predictions, ensuring that generated frames maintain logical temporal consistency.
- Optical Flow Algorithms: By analyzing the displacement of pixels between frames, these methods allow the system to simulate natural motion.
- Neural Rendering: AI enhances the synthesized video by applying realistic lighting, shading, and depth adjustments to make the animation more lifelike.
By combining these advanced techniques, image-to-video synthesis techniques can produce high-quality animations that closely resemble real-life motion, making them invaluable for industries such as entertainment, advertising, and virtual reality.
Common Image-to-Video Synthesis Techniques
Several image-to-video synthesis techniques have been developed to generate realistic motion from static images. These methods leverage AI, deep learning, and computer vision to create smooth and visually appealing video sequences. Below are some of the most widely used techniques:

1. Frame Interpolation
Frame interpolation involves generating intermediate frames between two images to create smooth motion. AI-powered models analyze motion trajectories and predict missing frames, allowing for fluid transitions. This technique is commonly used in slow-motion video generation and video frame rate enhancement.
2. Generative Adversarial Networks (GANs)
GANs have significantly advanced image-to-video synthesis techniques by enabling the generation of highly realistic motion. These networks consist of two components:
- Generator: Creates new video frames based on the input image.
- Discriminator: Evaluates whether the generated frames are realistic or not.
Through continuous training, GANs improve motion synthesis, making them widely used in deepfake technology, animation, and video content creation.
3. Neural Rendering
Neural rendering combines AI-based image analysis with traditional rendering techniques to generate videos with photorealistic details. This method enhances animations by adjusting lighting, shading, and depth, creating lifelike visuals.
4. Optical Flow-Based Methods
Optical flow techniques analyze the pixel displacement between frames to estimate motion. By predicting how pixels shift over time, these methods enable AI to synthesize realistic video sequences. Optical flow-based image-to-video synthesis techniques are commonly applied in motion tracking, video stabilization, and animation.
5. Motion Transfer and Keyframe Animation
Motion transfer techniques allow AI to apply movement patterns from a reference video onto a still image. Keyframe animation, on the other hand, uses manually defined motion points to generate video sequences. These approaches are widely used in deepfake applications, virtual avatars, and animated character generation.
Each of these image-to-video synthesis techniques plays a crucial role in different industries, from entertainment and gaming to marketing and AI-powered video automation. As AI continues to evolve, these methods are expected to become even more refined, enabling more realistic and efficient video synthesis.
Applications of Image-to-Video Synthesis
The advancement of image-to-video synthesis techniques has led to a wide range of applications across multiple industries. From entertainment to scientific research, these techniques are revolutionizing how static images are transformed into dynamic visuals. Below are some of the most impactful applications:

1. Film and Animation
The entertainment industry extensively uses image-to-video synthesis techniques for animation, visual effects (VFX), and content generation. AI-powered motion synthesis helps create lifelike animations, automate in-between frame generation, and enhance CGI effects in movies and video production.
2. Marketing and Advertising
Marketers and advertisers leverage AI-generated videos to create engaging promotional content. By transforming product images into short animations, brands can produce eye-catching advertisements, social media content, and interactive product demonstrations with minimal effort.
3. Video Game Development
In gaming, image-to-video synthesis techniques assist in character animation, background motion, and cutscene generation. AI-generated animations allow developers to create realistic movements for non-playable characters (NPCs) and dynamic environments without manually animating every frame.
4. Scientific Research and Medical Imaging
Medical imaging and scientific visualization benefit greatly from image-to-video synthesis techniques. These methods help generate animated models from MRI and CT scan images, providing better insights for diagnosis, treatment planning, and medical education. In scientific research, AI-driven video synthesis is used for simulations and data visualization.
5. Social Media and Content Creation
Content creators and influencers use AI-based video synthesis to animate still images, generate deepfake videos, and create engaging social media content. Platforms like TikTok, Instagram, and YouTube utilize AI tools to offer users creative ways to transform images into short videos with added effects and motion.
6. Virtual Reality (VR) and Augmented Reality (AR)
VR and AR applications use image-to-video synthesis techniques to create immersive experiences. AI-driven motion synthesis enhances virtual avatars, interactive 3D models, and AR overlays, making virtual environments more dynamic and interactive.
7. Historical and Cultural Preservation
AI-powered video synthesis is being used to bring historical figures and cultural artifacts to life. Museums and educational institutions utilize this technology to animate ancient paintings, historical photographs, and cultural exhibits, providing an interactive learning experience.
The rapid evolution of image-to-video synthesis techniques continues to open new possibilities across various domains. As AI improves, these applications will become even more advanced, enabling faster, more realistic, and creative video generation solutions.
Future of Image-to-Video Synthesis
The future of image-to-video synthesis techniques is filled with groundbreaking possibilities as artificial intelligence continues to evolve. With advancements in deep learning, motion prediction, and neural rendering, these techniques are expected to revolutionize digital content creation, automation, and real-time video generation.

1. Improved Realism with AI and Deep Learning
As AI models become more sophisticated, image-to-video synthesis techniques will achieve even greater levels of realism. Generative Adversarial Networks (GANs) and neural rendering technologies will enable highly detailed animations, ensuring natural motion, accurate physics, and lifelike textures. Future AI models will reduce artifacts, enhance frame consistency, and improve motion precision.
2. Real-Time Video Synthesis
One of the most exciting developments in image-to-video synthesis techniques is the ability to generate videos in real-time. This advancement will significantly impact virtual reality (VR), augmented reality (AR), and live-streaming applications, allowing users to animate images instantly and interact with AI-generated content seamlessly.
3. Increased Automation in Content Creation
The automation of video generation will reduce the need for manual animation and video editing. AI-powered synthesis will allow businesses, marketers, and content creators to produce high-quality animations with minimal effort. Personalized and AI-generated video advertisements, social media content, and even full-length animations could become the norm.
4. Ethical Considerations and Deepfake Detection
With the rise of deepfake technology, ethical concerns surrounding AI-generated videos are increasing. Future image-to-video synthesis techniques will likely include advanced deepfake detection algorithms to distinguish authentic content from AI-generated videos.
5. Enhanced Virtual Avatars and AI-Driven Interactions
The evolution of AI-generated avatars will transform industries such as customer service, gaming, and virtual meetings. Image-to-video synthesis techniques will enable highly interactive, emotion-responsive avatars capable of real-time conversation, facial expression synthesis, and personalized video communication.
6. Expanding Applications in Education and Healthcare
Educational institutions and medical professionals will benefit from advanced AI-generated video content. AI-powered visualizations will enhance remote learning, animated historical reconstructions, and medical simulations for training and diagnosis.
The rapid progress in image-to-video synthesis techniques will continue to push the boundaries of AI-generated content. With ongoing research and ethical advancements, this technology is set to redefine video production, digital interaction, and immersive storytelling in the coming years.
Challenges and Limitations of Image-to-Video Synthesis
Despite the rapid advancements in image-to-video synthesis techniques, several challenges and limitations hinder their full potential. These issues range from technical constraints to ethical concerns, affecting the quality, reliability, and responsible use of AI-generated videos.
1. Lack of Realism and Artifacts
Even with sophisticated AI models, achieving perfect realism remains a challenge. AI-generated videos often suffer from:
- Motion Artifacts: Inconsistent or unnatural motion transitions.
- Blur and Distortion: Loss of detail, especially in complex textures and lighting conditions.
- Frame Inconsistency: Flickering between frames, making the video appear unstable.
Enhancing realism requires continuous improvements in deep learning models, better motion estimation, and refined neural rendering techniques.
2. Computational and Hardware Limitations
High-quality image-to-video synthesis techniques demand significant computational power and advanced hardware, such as GPUs and AI accelerators. This makes real-time video synthesis challenging, especially for individuals or small businesses with limited resources. Cloud-based AI solutions and optimized models may help reduce these constraints in the future.
3. Data Dependency and Training Challenges
AI models require vast amounts of high-quality training data to generate accurate motion synthesis. Challenges include:
- Data Bias: If training datasets are unbalanced, AI may generate unrealistic or biased video content.
- High Training Costs: Developing powerful AI models demands expensive computational resources and extensive datasets.
- Limited Generalization: AI struggles to generate natural movement for unseen objects or complex real-world scenarios.
Improving dataset diversity and refining AI training methodologies can help overcome these issues.
4. Ethical and Deepfake Concerns
One of the biggest concerns surrounding image-to-video synthesis techniques is their misuse in creating deepfake content. AI-generated videos can be used for:
- Misinformation and Fake News: Altering real events or creating misleading narratives.
- Privacy Violations: Unauthorized synthesis of personal images into videos.
- Fraud and Identity Theft: AI-generated videos mimicking real people for malicious purposes.
To combat these risks, deepfake detection systems, digital watermarks, and AI regulations are being developed.
5. Lack of User Control and Creativity Constraints
While AI-powered synthesis simplifies video creation, it also limits user control. Many AI models generate videos based on predefined patterns, restricting artistic flexibility. Fine-tuning motion dynamics, emotions, and complex interactions often require manual intervention or additional tools.
6. Real-Time Performance Challenges
Real-time image-to-video synthesis techniques face latency issues, especially for interactive applications like virtual reality (VR) and augmented reality (AR). Reducing processing delays while maintaining video quality is an ongoing challenge for AI researchers.
While image-to-video synthesis techniques offer immense potential, overcoming these challenges is crucial for widespread adoption. Addressing realism issues, improving computational efficiency, ensuring ethical AI usage, and enhancing user control will drive future advancements in this field. As technology evolves, these limitations will gradually diminish, leading to more sophisticated and responsible AI-generated video solutions.
Conclusion
The evolution of image-to-video synthesis techniques is transforming the way static images are brought to life. By leveraging AI, deep learning, and advanced motion prediction models, these techniques are enabling the creation of high-quality, realistic video content with minimal human intervention. From entertainment and gaming to healthcare and marketing, the applications of this technology are expanding rapidly.
As AI continues to improve, future image-to-video synthesis techniques will offer even greater realism, automation, and accessibility. Real-time video generation, enhanced deepfake detection, and AI-driven content creation will revolutionize digital media. However, ethical considerations and responsible AI usage will remain crucial in shaping the future of synthesized video content.
With endless possibilities on the horizon, image-to-video synthesis techniques are set to redefine animation, storytelling, and digital communication, making AI-powered video generation a fundamental tool in various industries.