Image-to-Video Generation Methods
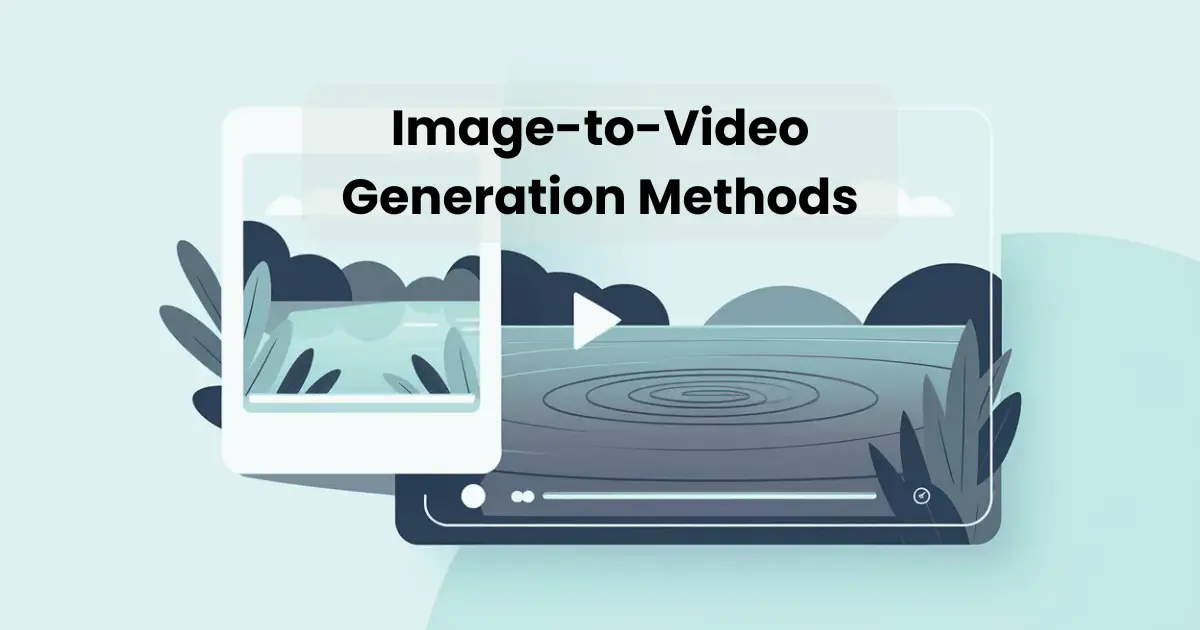
Contents
- 1 What is Image-to-Video Generation?
- 2 Why is Image-to-Video Generation Important?
- 3 Popular Image-to-Video Generation Methods
- 4 AI-Powered Image-to-Video Generation Tools
- 5 How to Choose the Right Image-to-Video Generation Method
- 6 Challenges in Image-to-Video Generation
- 6.1 1. Lack of Realistic Motion
- 6.2 2. Limited Understanding of Depth and Perspective
- 6.3 3. High Computational Requirements
- 6.4 4. Data Dependency and Training Limitations
- 6.5 5. Artifacts and Distortions
- 6.6 6. Ethical and Copyright Concerns
- 6.7 7. Lack of Customization in AI Models
- 6.8 8. Real-Time Processing Challenges
- 7 Future Trends in Image-to-Video Generation
- 7.1 1. Improved Motion Prediction and Realism
- 7.2 2. Real-Time Image-to-Video Generation
- 7.3 3. Higher-Resolution and Ultra-Realistic Videos
- 7.4 4. AI-Generated Storytelling and Content Creation
- 7.5 5. Personalized and Interactive AI Video Creation
- 7.6 6. Ethical and Regulatory Developments
- 7.7 7. Integration with Cloud and Edge Computing
- 7.8 8. AI-Human Collaboration in Video Production
- 7.9 Conclusion
In recent years, image-to-video generation methods have revolutionized digital content creation. This innovative technology transforms static images into dynamic videos, enhancing storytelling, marketing, and animation. With advancements in artificial intelligence, image-to-video generation methods have become more efficient, producing lifelike motion and seamless transitions. As industries increasingly rely on automated video generation, understanding the available techniques, tools, and applications is essential. This article explores various image-to-video generation methods, their importance, challenges, and future trends shaping this evolving field.
What is Image-to-Video Generation?
Image-to-video generation is the process of transforming static images into dynamic video sequences by applying animation techniques, artificial intelligence, or motion interpolation. This technology enables the creation of smooth transitions, realistic motion, and visually engaging animations from a single image or a set of images.
Modern image-to-video generation methods leverage deep learning, neural networks, and generative models to predict and generate intermediate frames, simulating motion naturally. These techniques are widely used in animation, filmmaking, advertising, and AI-driven content creation. By automating video production, they enhance efficiency, reduce manual effort, and open new creative possibilities for digital media.
Why is Image-to-Video Generation Important?

1. Enhancing Visual Storytelling
Image-to-video generation methods allow creators to bring static images to life, making visual content more engaging and immersive. This is especially useful in filmmaking, animation, and digital marketing, where dynamic visuals capture attention more effectively than still images.
2. Automating Content Creation
With advancements in AI, image-to-video generation reduces the need for manual animation and editing. Businesses and content creators can generate high-quality videos quickly, saving time and resources. AI-driven tools streamline production workflows, making video creation accessible to everyone.
3. Improving Marketing and Advertising
Brands use image-to-video generation methods to create eye-catching promotional content. Animated product visuals, motion graphics, and AI-generated advertisements enhance user engagement, increasing conversion rates and brand awareness.
4. Advancing AI and Deep Learning Research
AI-powered image-to-video generation contributes to research in computer vision, deep learning, and synthetic media. Technologies like Generative Adversarial Networks (GANs) and motion prediction models push the boundaries of realistic video synthesis.
5. Expanding Applications in Various Industries
From healthcare to education, image-to-video generation methods serve multiple industries. Medical imaging benefits from animated visualizations, while educational content becomes more interactive with AI-generated instructional videos.
6. Revolutionizing Social Media and Entertainment
Social media platforms and entertainment industries rely on image-to-video generation for dynamic content creation. Short videos, GIFs, and AI-generated clips enhance user experience, making content more shareable and engaging.
As AI continues to evolve, image-to-video generation methods will play a crucial role in reshaping digital media, offering endless possibilities for creativity and automation.
Popular Image-to-Video Generation Methods
Various image-to-video generation methods are used to create seamless motion from static images. These techniques range from traditional animation approaches to advanced AI-driven models. Below are some of the most widely used methods:

1. AI-Based Image-to-Video Generation
Artificial intelligence has revolutionized image-to-video generation methods, making them more efficient and realistic. AI-powered techniques analyze an image and predict motion patterns to create a smooth video sequence. Some of the most advanced AI approaches include:
- Generative Adversarial Networks (GANs): GANs generate high-quality videos by training two neural networks—one creates the video while the other evaluates its realism. This results in highly lifelike motion generation.
- Diffusion Models: These AI models generate intermediate frames by predicting motion and filling in missing details, producing smooth and natural video transitions.
- Neural Radiance Fields (NeRF): This method reconstructs 3D scenes from images, allowing for realistic perspective changes in videos.
2. Traditional Animation Techniques
Before AI, animation techniques were the primary way to create videos from images. These methods are still widely used in creative industries:
- Frame-by-Frame Animation: Artists create multiple images with slight variations, which are then played in sequence to create movement.
- Stop Motion Animation: Objects or images are physically manipulated and captured in small increments to simulate motion when played as a video.
- Time-Lapse Photography: A sequence of images is taken over time and then compiled into a fast-motion video, commonly used for natural scenery, cityscapes, or scientific observations.
3. Motion Interpolation
Motion interpolation, also known as frame interpolation, generates additional frames between two existing frames to create smoother motion. AI-driven tools like FlowFrames and RIFE (Real-Time Intermediate Flow Estimation) predict movement and fill in gaps, enhancing video smoothness.
4. Keyframe Animation
Keyframe animation involves setting key points in a sequence where motion changes occur. Animation software then generates intermediate frames between keyframes, creating fluid movement. This method is commonly used in digital animation, video editing, and game design.
5. Generative Adversarial Networks (GANs) for Face Animation
GAN-based tools, such as Deep Nostalgia and D-ID, create lifelike animations from static portraits. These tools analyze facial features and simulate expressions, eye movement, and head tilts, bringing historical figures and old photos to life.
Each of these image-to-video generation methods has unique advantages, depending on the required level of realism, automation, and artistic control. As technology advances, AI-powered techniques continue to dominate, offering new possibilities in digital content creation.
AI-Powered Image-to-Video Generation Tools
With the rise of artificial intelligence, various AI-powered image-to-video generation tools have made it easier to create realistic and dynamic video content from static images. These tools utilize deep learning, generative models, and motion prediction techniques to generate high-quality videos with minimal manual effort. Here are some of the most popular tools available today:

1. RunwayML
RunwayML is a powerful AI-driven tool that enables users to generate videos from images using advanced machine learning models. It provides features such as motion interpolation, background removal, and AI-generated animations. The platform is user-friendly and widely used by content creators and filmmakers.
2. Pika Labs
Pika Labs specializes in AI-driven animation, allowing users to convert images into videos effortlessly. Its models focus on smooth motion synthesis, making it ideal for generating artistic and promotional content.
3. D-ID
D-ID is known for its AI face animation technology, which can animate portraits by adding realistic facial expressions and head movements. This tool is often used in marketing, social media, and historical image restoration.
4. Deep Nostalgia
Developed by MyHeritage, Deep Nostalgia animates old photos by applying deep learning models to simulate blinking, smiling, and head tilts. It is mainly used for bringing historical images to life.
5. Kaiber AI
Kaiber AI is an advanced image-to-video generation platform that allows users to create stunning AI-generated videos with artistic effects. It is commonly used in digital marketing and music video production.
6. Deep Dream Generator
Deep Dream Generator is an AI-based tool that transforms static images into dreamlike, moving visuals using deep neural networks. It is often used for artistic video creation and experimental animations.
7. Artbreeder Video
Artbreeder utilizes AI-powered image morphing to generate animated sequences by blending images with smooth transitions. It is a useful tool for concept artists and designers looking to visualize evolving artwork.
8. Google’s Phenaki
Google’s Phenaki is a state-of-the-art AI model that generates long-duration videos from a single image and text descriptions. It creates realistic animations and is expected to be widely used in future AI-generated content.
9. Synthesia
Synthesia is an AI-powered video generation platform that creates animated avatars and explainer videos from images and text. It is primarily used in corporate presentations, e-learning, and marketing.
10. HeyGen
HeyGen allows users to generate AI-driven image-to-video animations with smooth motion and customizable features. It is designed for social media content creators and businesses looking to automate video production.
These AI-powered image-to-video generation tools have significantly advanced content creation, making it more accessible for artists, marketers, and developers. As AI continues to improve, these tools will play a crucial role in shaping the future of digital media and animation.
How to Choose the Right Image-to-Video Generation Method
Selecting the best image-to-video generation method depends on various factors, including the level of realism required, the purpose of the video, and the available tools. Here are the key considerations to help you choose the right method:

1. Define Your Purpose
Different image-to-video generation methods cater to different needs. Consider the following applications:
- Marketing & Advertising → AI-powered tools like RunwayML and Kaiber AI for engaging promotional videos.
- Art & Creativity → Generative models such as Deep Dream Generator for stylized animations.
- Historical & Personal Use → Face animation tools like Deep Nostalgia or D-ID for animating old photographs.
2. Consider the Level of Realism
- Highly realistic motion → AI-based methods using GANs or NeRF models.
- Smooth transitions with limited frames → Motion interpolation techniques.
- Artistic and stylized videos → Deep learning-based style transfer tools.
3. Evaluate Technical Requirements
- AI-Powered Tools → Require high-performance GPUs or cloud-based services.
- Traditional Animation → Demands artistic skills and manual frame creation.
- Motion Interpolation → Needs minimal computing power but works best with high-resolution images.
4. Ease of Use vs. Customization
- User-friendly tools → Platforms like Synthesia and HeyGen offer simple, automated solutions.
- Advanced customization → Software like RunwayML provides more control over motion and effects.
5. Cost & Accessibility
- Free or Freemium Options → Deep Nostalgia, D-ID, and RunwayML have free plans with limited features.
- Premium Tools → Synthesia, Kaiber AI, and Phenaki offer advanced AI capabilities but require subscriptions.
6. Scalability & Integration
For businesses and large-scale projects, choose methods that integrate with existing workflows:
- APIs & Automation → AI tools with API support for bulk processing.
- Cloud-Based Solutions → Platforms like Pika Labs allow remote processing for seamless content creation.
By considering these factors, you can select the most suitable image-to-video generation method for your needs. Whether for personal projects or professional applications, choosing the right approach ensures efficiency, creativity, and high-quality results.
Challenges in Image-to-Video Generation
Despite advancements in AI and deep learning, image-to-video generation still faces several challenges. These obstacles affect the quality, realism, and efficiency of the generated videos. Below are some of the major challenges:

1. Lack of Realistic Motion
Many image-to-video generation methods struggle to produce natural motion. AI models may fail to predict accurate movements, leading to:
- Jerky or unnatural transitions between frames.
- Distorted body parts in facial animation tools.
- Inconsistent motion flow, especially in complex scenes.
2. Limited Understanding of Depth and Perspective
Some AI models lack a proper understanding of depth perception, leading to:
- Flat or unrealistic 3D effects.
- Incorrect object scaling and positioning in motion synthesis.
- Difficulties in handling multiple perspective shifts.
3. High Computational Requirements
Many AI-powered image-to-video generation tools require powerful hardware, such as GPUs and cloud-based servers. Challenges include:
- Expensive hardware for high-quality output.
- Slow processing times for complex animations.
- Limited accessibility for users with low-end devices.
4. Data Dependency and Training Limitations
AI models need vast datasets to improve video realism. However, challenges arise due to:
- Bias in training data, leading to inaccurate animations.
- Difficulty in animating unseen objects that were not in the dataset.
- Limited generalization, making AI struggle with unique or creative inputs.
5. Artifacts and Distortions
Many image-to-video generation methods suffer from unwanted artifacts, such as:
- Blurry or pixelated frames in AI-generated videos.
- Weird distortions in facial expressions and body movements.
- Flickering effects due to inconsistent frame generation.
6. Ethical and Copyright Concerns
AI-generated videos raise legal and ethical concerns, such as:
- Deepfake misuse, leading to misinformation and privacy violations.
- Intellectual property issues, as AI may replicate copyrighted styles.
- Consent concerns, especially when animating real people’s images.
7. Lack of Customization in AI Models
While AI tools automate image-to-video generation, they often provide limited control over:
- Motion paths and transitions.
- Facial expressions and emotions.
- Camera angles and scene composition.
8. Real-Time Processing Challenges
For applications requiring real-time image-to-video generation, challenges include:
- Latency issues, making live AI-generated videos difficult.
- Resource-intensive computations, leading to slow response times.
- Synchronization problems, especially for interactive applications.
While image-to-video generation methods have made significant progress, these challenges highlight areas for further improvement. Future developments in AI, computing power, and data training will help overcome these limitations, making image-to-video generation more seamless and realistic.
Future Trends in Image-to-Video Generation
As artificial intelligence and machine learning continue to evolve, image-to-video generation methods are expected to become more advanced, efficient, and accessible. Emerging technologies and innovations will enhance realism, creativity, and automation in video generation. Below are the key future trends in this field:

1. Improved Motion Prediction and Realism
Future AI-powered image-to-video generation tools will focus on generating more natural and fluid motion by:
- Utilizing neural radiance fields (NeRFs) to improve depth perception.
- Enhancing GAN-based models to produce realistic animations.
- Incorporating physics-based simulations for more accurate movement.
2. Real-Time Image-to-Video Generation
Advancements in computational power and AI models will enable real-time video generation, benefiting industries such as:
- Gaming – AI-driven animation for dynamic character movements.
- Virtual Reality (VR) and Augmented Reality (AR) – Instant video synthesis for immersive experiences.
- Live Streaming – AI-generated animations reacting in real time to user inputs.
3. Higher-Resolution and Ultra-Realistic Videos
AI will continue to push the boundaries of image-to-video generation with:
- 4K and 8K video generation for professional-grade content.
- Advanced super-resolution techniques to enhance image clarity.
- More detailed textures, lighting, and shading for photorealistic results.
4. AI-Generated Storytelling and Content Creation
In the future, AI models will not only generate videos but also create narratives and storytelling elements, such as:
- AI-directed animations that include automated scene transitions and camera movements.
- Character-driven animations, where AI animates digital avatars with lifelike expressions.
- Personalized content generation, allowing users to create custom video stories.
5. Personalized and Interactive AI Video Creation
Future image-to-video generation methods will be more interactive, enabling:
- Voice and text-driven animations, where users can describe a scene, and AI animates it.
- Gesture-based video synthesis, allowing motion control using body movements.
- AI-powered customization, letting users adjust motion paths, styles, and effects.
6. Ethical and Regulatory Developments
As AI-generated videos become mainstream, new regulations and ethical guidelines will be introduced to address:
- Deepfake detection and authentication tools to prevent misuse.
- Copyright and intellectual property rights for AI-generated content.
- Transparent AI training models, ensuring fair and unbiased content generation.
7. Integration with Cloud and Edge Computing
Cloud-based and edge computing solutions will make image-to-video generation faster and more efficient by:
- Reducing processing time for large-scale video generation.
- Allowing AI video synthesis on mobile and low-power devices.
- Providing seamless cloud-based storage and sharing options.
8. AI-Human Collaboration in Video Production
Rather than replacing human creativity, AI will act as a collaborative tool, allowing:
- Artists and filmmakers to generate quick animations as a base for further refinements.
- Automated editing tools that assist in post-production tasks.
- AI-assisted filmmaking, where directors can preview AI-generated scenes before filming.
The future of image-to-video generation is driven by AI advancements, real-time processing, and enhanced realism. As these technologies evolve, they will revolutionize industries such as entertainment, marketing, education, and virtual experiences. The coming years will bring faster, smarter, and more creative ways to transform static images into dynamic videos.
Conclusion
The evolution of image-to-video generation methods has transformed how static visuals are brought to life. With advancements in artificial intelligence, deep learning, and computer vision, the process of converting images into dynamic videos has become more efficient and accessible. From AI-powered tools to traditional animation techniques, these methods offer endless possibilities for content creators, businesses, and industries.
Despite its rapid growth, image-to-video generation still faces challenges such as realistic motion synthesis, computational requirements, and ethical concerns. However, future trends indicate significant improvements, including real-time processing, enhanced personalization, and ultra-realistic video generation. As AI continues to evolve, the quality, speed, and accuracy of image-to-video generation will improve, making it a valuable tool for storytelling, marketing, and creative industries.
Ultimately, the future of image-to-video generation lies in seamless AI-human collaboration, where technology enhances creativity rather than replacing it. Whether for professional filmmaking, social media content, or virtual experiences, image-to-video generation methods will continue to shape the way we interact with visual media.