New Image Processing Models | Challenges and Limitations
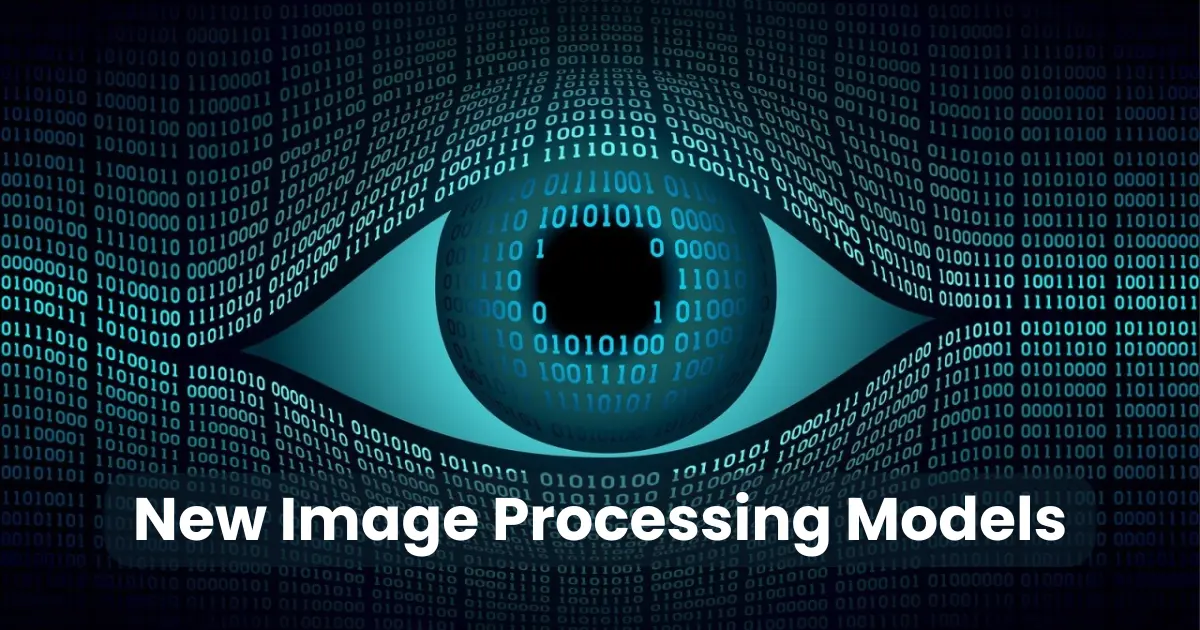
Contents
- 1 Evolution of Image Processing Techniques
- 2 Key Features of New Image Processing Models
- 3 Applications in Various Industries
- 4 Advantages Over Traditional Image Processing
- 5 Challenges and Limitations
- 6 Future of Image Processing Models
- 6.1 1. Integration of AI and Edge Computing
- 6.2 2. Enhanced Real-Time Processing and Low Latency
- 6.3 3. Continuous Improvement in Accuracy and Generalization
- 6.4 4. Democratization of AI and Image Processing
- 6.5 5. Explainability and Ethical Considerations
- 6.6 6. Fusion of Multi-Modal Data
- 6.7 7. Automation of Image Annotation
- 7 Conclusion
New image processing models are revolutionizing how we analyze, enhance, and interpret digital images. These advanced models, powered by artificial intelligence and deep learning, significantly improve the quality of image recognition, noise reduction, and feature extraction. Unlike traditional methods, these models automate tasks and offer more precise results. As a result, industries such as healthcare, security, and entertainment are seeing major improvements in their visual data processing capabilities. In this article, we will explore how new image processing models work, their applications, and the future advancements that will continue shaping the field.
What Are New Image Processing Models?
New image processing models are advanced computational frameworks designed to enhance, analyze, and manipulate digital images using cutting-edge technologies. Unlike traditional image processing techniques, which often rely on manual algorithms, these models incorporate artificial intelligence (AI), machine learning, and deep learning to automate image analysis. By leveraging neural networks, these models can efficiently recognize patterns, classify objects, and improve image quality. The result is a more intelligent and adaptable system capable of handling complex image-related tasks with higher accuracy and speed. These models are becoming increasingly important in industries like healthcare, security, entertainment, and beyond, where the need for fast and precise image processing is critical.
Evolution of Image Processing Techniques
Image processing has undergone significant transformations, evolving from simple manual techniques to highly sophisticated AI-powered models. Initially, image processing techniques were rule-based, focusing on basic tasks such as edge detection, filtering, and contrast enhancement. These early methods relied on straightforward algorithms that could only handle simple operations, such as adjusting brightness or removing noise.
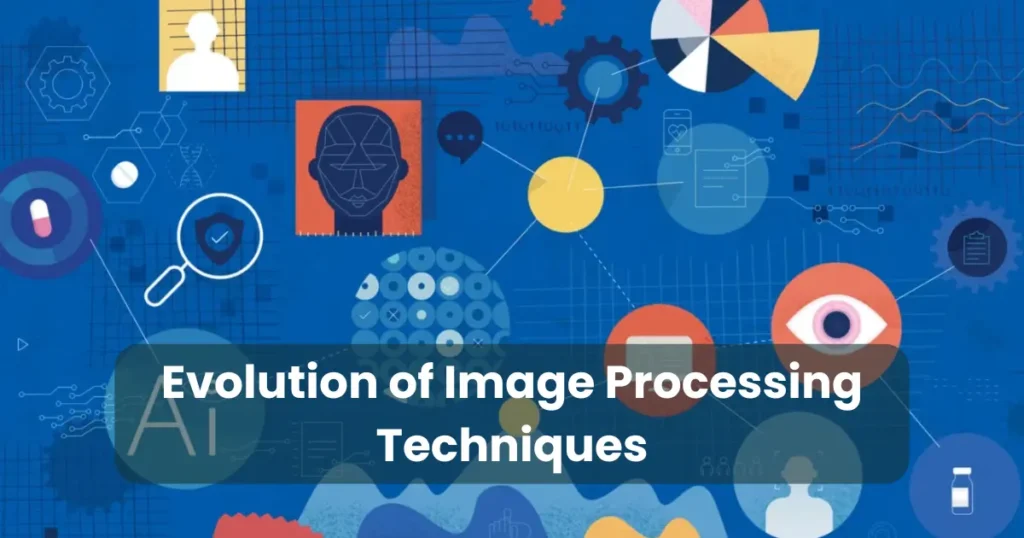
As computing power advanced, machine learning techniques began to emerge, allowing for more complex image analysis tasks. For instance, algorithms started to automate processes like feature extraction and object recognition, making it possible to analyze larger datasets without manual intervention.
In the past decade, the rise of deep learning has marked a revolutionary shift. Deep learning models, particularly Convolutional Neural Networks (CNNs), are now used for tasks like object detection, image segmentation, and even generating high-quality images from low-resolution inputs. These models are able to learn patterns from vast amounts of data, improving their performance over time. Unlike traditional methods, which required human-defined rules, deep learning models are capable of identifying patterns and making decisions independently.
Today, new image processing models leverage the power of AI, deep learning, and neural networks to achieve unprecedented accuracy and speed in real-time processing. This progression has allowed industries to adopt image processing techniques for more practical and impactful applications, ranging from medical imaging to autonomous driving, and beyond.
Key Features of New Image Processing Models
New image processing models come equipped with advanced features that set them apart from traditional techniques. These features enable them to handle more complex tasks with greater efficiency and accuracy. Here are some of the key characteristics of these models:
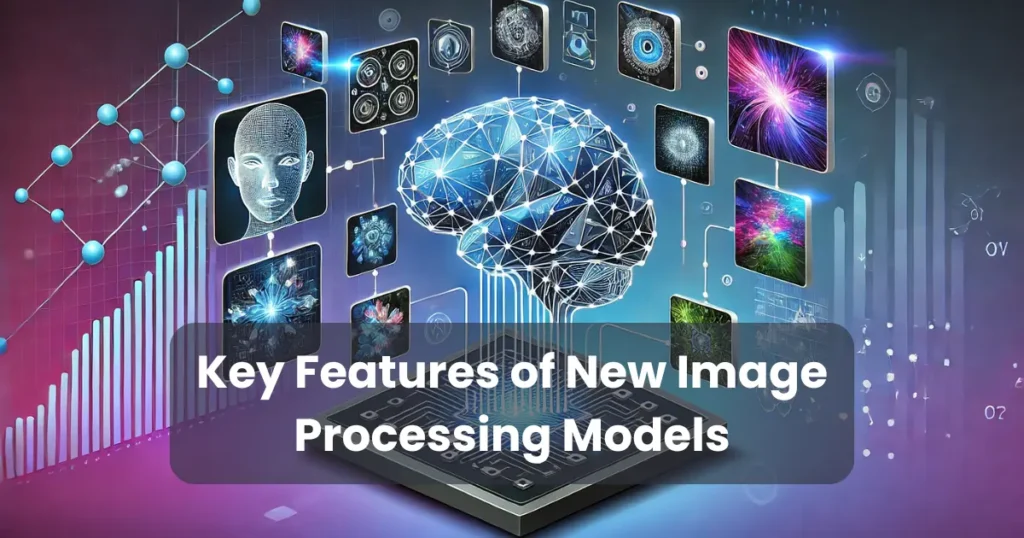
Deep Learning Integratin
Deep learning, especially through Convolutional Neural Networks (CNNs), is central to new image processing models. These networks automatically learn to recognize patterns in data, eliminating the need for manually defined rules. By processing vast amounts of image data, deep learning models can detect intricate patterns that would otherwise be difficult to identify using conventional methods.
Real-Time Processing
One of the standout features of modern image processing models is their ability to process images in real-time. This is achieved through powerful GPUs and optimized algorithms that allow for rapid computations. Real-time processing is essential for applications like video surveillance, autonomous vehicles, and medical imaging, where immediate analysis and decision-making are crucial.
Noise Reduction and Image Enhancement
New models excel in reducing noise and improving image clarity. Advanced filtering techniques can eliminate distortions, ensuring that the processed images are sharp and clear. For example, super-resolution algorithms can enhance low-resolution images, making them usable for detailed analysis or visualization.
Automated Image Recognition
These models are equipped with highly sophisticated image recognition capabilities. They can automatically detect and classify objects within an image, even when the objects are hidden or partially obscured. This feature is particularly useful in fields such as security, healthcare, and e-commerce, where identifying specific objects is critical.
Adaptive Learning
New image processing models continuously learn and adapt as they are exposed to new data. Unlike traditional models, which are static and require manual updates, these models improve over time through a process known as transfer learning. This ability to adapt ensures they remain effective, even as the types of images or objects they analyze evolve.
Multi-Scale Analysis
Many new image processing models perform multi-scale analysis, which means they can examine an image at different levels of resolution and context. This enables a more thorough analysis, allowing the model to detect details that may be missed in a single-scale approach.
Semantic Segmentation
Semantic segmentation allows for the classification of different parts of an image into distinct categories. This feature is vital in applications like autonomous driving, where the system must differentiate between pedestrians, vehicles, roads, and other objects in the environment.
These key features make new image processing models more efficient and versatile than traditional methods. As technology continues to evolve, these models will only become more powerful, enabling more precise and faster image analysis across various industries.
Applications in Various Industries
New image processing models are not only advancing the field of digital imaging but are also making significant contributions to a wide range of industries. Their ability to process, analyze, and interpret images with high accuracy has led to innovative solutions in multiple sectors. Here are some of the key applications of these models across various industries:
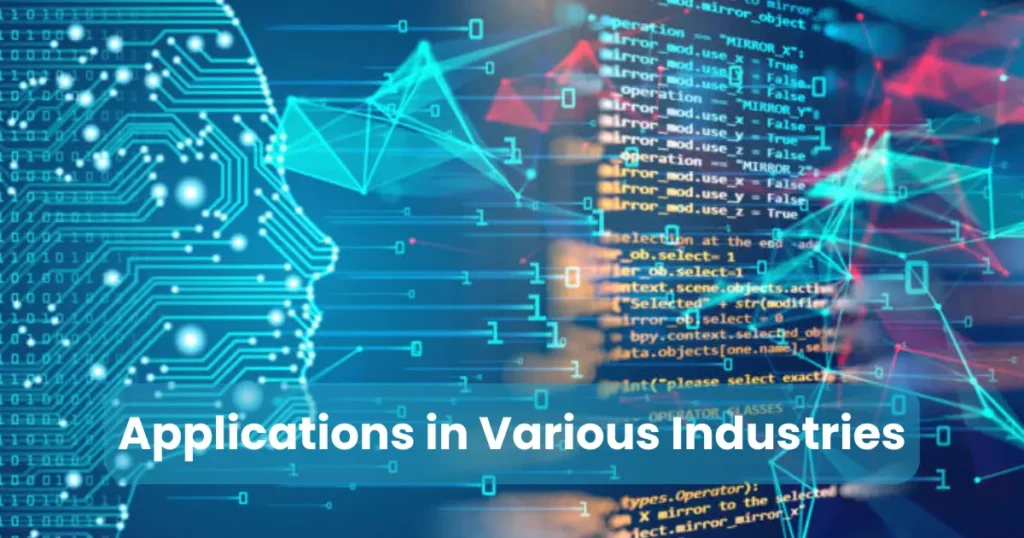
1. Healthcare
In healthcare, new image processing models are revolutionizing medical imaging. Advanced models assist in analyzing X-rays, CT scans, MRIs, and ultrasound images, helping healthcare professionals detect conditions like tumors, fractures, and organ abnormalities with greater precision. Deep learning models can automate diagnosis by identifying patterns that might be overlooked by human experts, leading to faster and more accurate results. Additionally, image processing techniques are essential in surgical planning and robot-assisted surgeries, where real-time image analysis ensures higher success rates.
2. Security and Surveillance
Security systems have greatly benefited from the evolution of image processing models. Facial recognition, object tracking, and anomaly detection are now automated tasks, enabling more effective monitoring in public spaces, airports, and other sensitive areas. These systems can process large amounts of visual data in real-time, enhancing security efforts by detecting potential threats faster than traditional methods. Furthermore, advanced models can also analyze footage from CCTV cameras to automatically identify suspicious activities, reducing human oversight and improving overall security.
3. Entertainment and Media
In the entertainment industry, image processing models are widely used for enhancing video quality, creating special effects, and improving CGI (computer-generated imagery). New models allow filmmakers to create hyper-realistic visuals by generating highly detailed textures and dynamic lighting effects. Video games also benefit from these advancements, as real-time rendering models enable more realistic environments and character animations. Additionally, new image processing techniques are used to restore old films, upscale low-resolution content, and create deepfake technology for visual storytelling.
4. Autonomous Vehicles
Autonomous vehicles rely heavily on image processing models for navigation and safety. These models enable self-driving cars to interpret data from cameras, lidar, and radar sensors, allowing the vehicle to identify pedestrians, other vehicles, road signs, and obstacles. By analyzing images and video feeds in real-time, these models ensure that autonomous vehicles can make immediate decisions, such as stopping for pedestrians or adjusting speed based on road conditions. As a result, image processing models play a crucial role in enhancing the safety and functionality of autonomous transportation.
5. Retail and E-Commerce
In retail and e-commerce, image processing models are transforming how businesses interact with customers. Models can automatically classify products, detect defects in items, and even help with inventory management by analyzing images of products. Visual search technology, powered by deep learning models, allows customers to search for products by uploading images, improving the online shopping experience. Additionally, these models are used to analyze consumer behavior through visual data, such as facial expressions and body language, to optimize marketing strategies and product placements.
6. Agriculture
In agriculture, image processing models help monitor crop health, detect diseases, and assess soil quality. By analyzing images captured through drones or satellites, these models can provide farmers with insights into the condition of their crops, enabling timely interventions. Furthermore, automated systems can identify weeds, pests, and other factors that affect crop yields, helping farmers increase productivity and reduce the use of pesticides. These applications contribute to more sustainable farming practices and better resource management.
7. Manufacturing and Quality Control
New image processing models are widely used in manufacturing for quality control and defect detection. These models can automatically inspect products during the production process to ensure they meet quality standards. By analyzing images of components or finished products, models can detect flaws, such as cracks or misalignments, that may be invisible to the human eye. This level of precision reduces waste, minimizes human error, and enhances product quality. Additionally, these models are used in predictive maintenance, analyzing visual data from equipment to predict failures before they occur.
8. Aerospace and Defense
In aerospace and defense, image processing models are applied for surveillance, reconnaissance, and object detection. Satellite and drone imagery are processed to provide real-time intelligence for military operations. These models can analyze vast amounts of visual data to identify potential threats, map terrain, and monitor enemy movements. In addition, image processing is essential for the development of advanced navigation systems used in unmanned aerial vehicles (UAVs) and spacecraft.
The versatility and precision of new image processing models have made them indispensable across a wide range of industries. By automating complex tasks and providing actionable insights, these models not only enhance operational efficiency but also drive innovation and new possibilities in fields such as healthcare, security, entertainment, and beyond.
Advantages Over Traditional Image Processing
New image processing models, especially those powered by artificial intelligence and deep learning, offer several advantages over traditional methods. These advantages make them far more effective, efficient, and versatile in handling complex image-related tasks. Here are some key benefits of using modern image processing models:
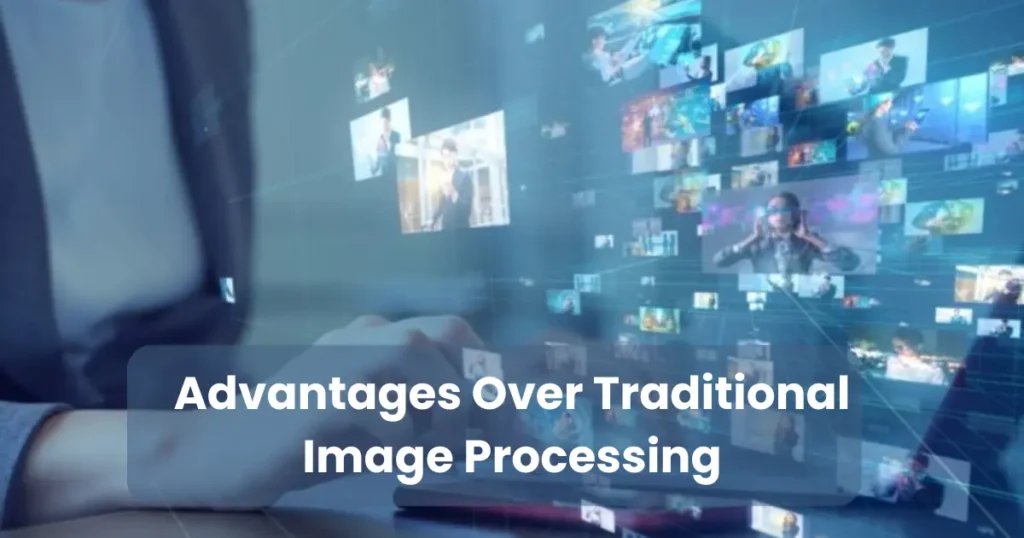
1. Higher Accuracy and Precision
One of the primary advantages of new image processing models is their ability to achieve higher accuracy and precision in image analysis. Traditional methods often require manual adjustments and predefined rules, which can be prone to human error. In contrast, deep learning models can automatically learn from vast amounts of data, allowing them to recognize complex patterns and deliver more accurate results, especially in tasks like object recognition and medical imaging.
2. Automation of Complex Tasks
Modern image processing models automate many complex and time-consuming tasks that would be labor-intensive using traditional methods. For example, instead of manually enhancing an image or identifying objects, these models can process images in real-time and autonomously detect features, classify objects, and improve image quality. This automation saves time and reduces the reliance on human input, allowing for faster decision-making and more efficient workflows.
3. Real-Time Processing
While traditional image processing methods often operate in batch processing, modern models are designed for real-time image analysis. This means they can process and interpret images instantly, making them ideal for applications like live surveillance monitoring, autonomous driving, and real-time medical diagnostics. Real-time processing is crucial in industries where rapid decision-making is needed, and new models excel in delivering this capability with low latency.
4. Adaptability and Continuous Learning
New image processing models have the ability to continuously learn and adapt based on new data. Unlike traditional methods, which require manual updates and fine-tuning, AI-powered models improve over time through processes like transfer learning. This adaptability makes them more robust and capable of handling a wider variety of images and evolving tasks. For example, as a model is exposed to new types of images or objects, it can automatically adjust and refine its algorithms for better performance.
5. Improved Noise Reduction and Image Enhancement
Traditional methods of noise reduction and image enhancement often involve filtering techniques that can lead to loss of details or introduce artifacts. In contrast, modern image processing models, especially those using deep learning, are better at enhancing image quality without sacrificing detail. These models can remove noise while preserving critical features, ensuring a clearer and more accurate final image. Techniques like super-resolution are also applied to improve the quality of low-resolution images.
6. Better Handling of Complex and Large Datasets
New image processing models are capable of handling large and complex datasets with ease. Traditional methods often struggle with large volumes of data or highly detailed images, leading to slower processing times and decreased accuracy. AI and machine learning models, on the other hand, are designed to handle massive datasets and can process high-resolution images more efficiently. This capability is especially valuable in fields like satellite imaging, medical imaging, and industrial applications, where large datasets are common.
7. Flexibility Across Different Domains
Modern image processing models are highly adaptable and can be applied to a wide range of domains without significant changes to the core algorithm. Whether it’s healthcare, security, entertainment, or agriculture, these models can be tailored to suit different needs and can handle various types of images, including 2D, 3D, and even video. Traditional methods, in contrast, often require custom solutions for each specific task, making them less versatile.
In summary, the advantages of new image processing models over traditional methods are clear. From higher accuracy and real-time processing to continuous learning and better adaptability, these modern models provide more effective solutions for a wide range of industries. Their ability to automate complex tasks, reduce human error, and scale efficiently positions them as a critical tool for the future of image analysis and interpretation.
Challenges and Limitations
While new image processing models offer numerous advantages, they also come with their own set of challenges and limitations. These can impact their effectiveness and the widespread adoption of these technologies. Here are some of the key challenges and limitations associated with modern image processing models:
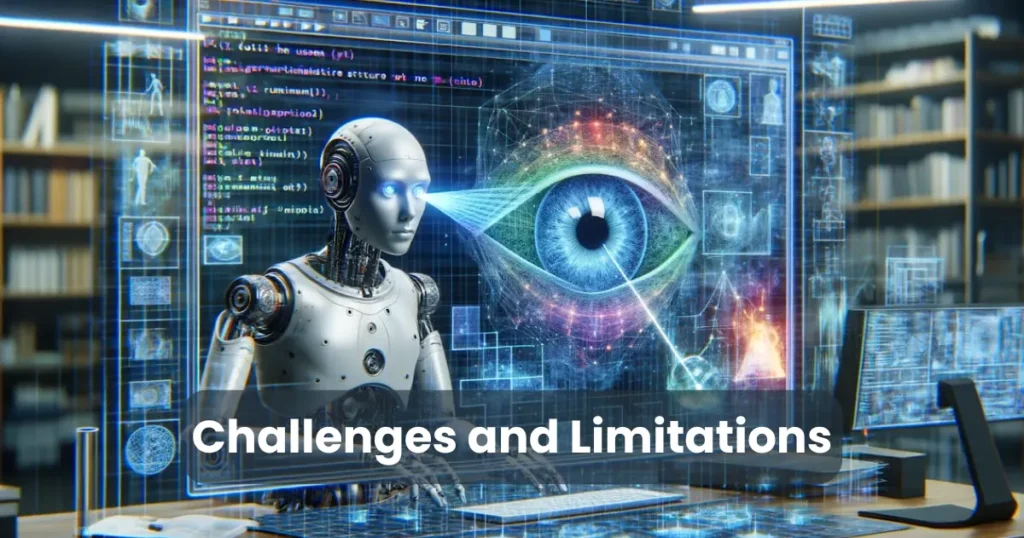
1. High Computational Requirements
One of the main challenges of new image processing models, particularly those based on deep learning, is the high computational power they require. Training deep learning models involves processing large datasets, which demands substantial computing resources, including powerful GPUs and specialized hardware. This can make the implementation of these models costly and difficult for smaller organizations with limited resources. Additionally, real-time processing at scale requires continuous access to high-performance infrastructure, which can add to operational expenses.
2. Data Quality and Quantity
For image processing models to perform at their best, they require large amounts of high-quality data. Collecting and labeling this data can be a time-consuming and expensive process. In many cases, the quality of the data directly impacts the model’s accuracy and performance. Poor-quality data, such as images with noise, blur, or incomplete annotations, can lead to inaccurate results and reduce the model’s effectiveness. Furthermore, certain industries or applications may lack sufficient datasets for training, making it difficult to create robust models.
3. Overfitting and Generalization Issues
Overfitting occurs when a model learns to recognize patterns specific to the training data but struggles to generalize to new, unseen data. This is a common issue in deep learning models, where the model becomes too specialized and fails to perform well on real-world data. While techniques like regularization and cross-validation are employed to mitigate overfitting, achieving the right balance between fitting the training data and maintaining the ability to generalize can be challenging. Models that are overfit can lead to incorrect predictions and limit their applicability in dynamic environments.
4. Lack of Transparency and Interpretability
Many new image processing models, particularly deep learning-based models, are often criticized for their lack of transparency and interpretability. These “black-box” models make it difficult to understand how they arrive at specific decisions. In critical applications like healthcare and security, this lack of explainability can be a significant drawback. Stakeholders may be hesitant to trust decisions made by a model without understanding the reasoning behind them. Research into explainable AI (XAI) is ongoing, but this remains a challenge for many industries relying on image processing models.
5. Ethical and Privacy Concerns
Image processing models, particularly those used in facial recognition and surveillance, have raised ethical and privacy concerns. The widespread use of these models can lead to potential misuse, such as unauthorized tracking of individuals or invasion of privacy. Additionally, issues related to bias and fairness can arise if the data used to train these models is not diverse enough. For example, biased training data can result in facial recognition systems that perform poorly for certain demographic groups, leading to inaccurate or discriminatory outcomes. Addressing these ethical concerns requires clear regulations and responsible model development.
While new image processing models offer groundbreaking capabilities, they also come with several challenges and limitations. High computational costs, data quality issues, overfitting concerns, lack of interpretability, and ethical considerations must be addressed to ensure the effective and responsible use of these models. Despite these challenges, ongoing advancements in technology and research continue to improve the capabilities and applicability of modern image processing models across various industries.
Future of Image Processing Models
The future of image processing models is incredibly promising, with advancements in artificial intelligence, machine learning, and deep learning paving the way for new capabilities and applications. As technology continues to evolve, the scope of image processing will expand, leading to innovations in various industries, from healthcare to entertainment. Here are some key trends and developments expected to shape the future of image processing models:
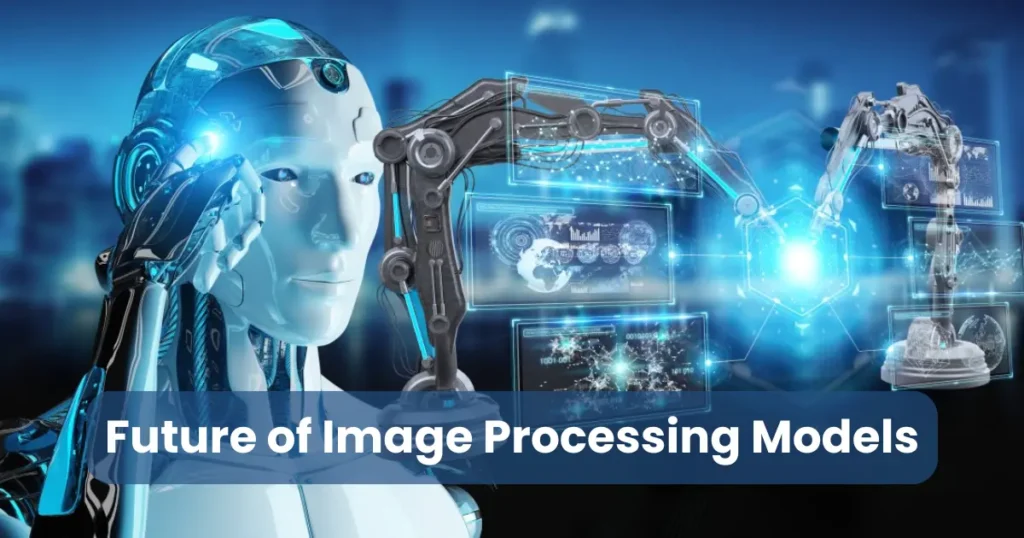
1. Integration of AI and Edge Computing
One of the most significant trends in the future of image processing models is the integration of AI with edge computing. Edge computing involves processing data closer to the source of the data, such as on the device itself, rather than relying on cloud servers. This allows for faster real-time image processing with lower latency, which is particularly crucial for applications like autonomous vehicles, real-time surveillance, and augmented reality (AR). As AI models become more efficient, they will be able to run on smaller devices with limited resources, reducing the need for centralized processing and enabling more localized, immediate analysis.
2. Enhanced Real-Time Processing and Low Latency
The demand for real-time processing is expected to grow across various industries. The future of image processing models will focus on minimizing latency, allowing for near-instantaneous results. This is especially important in sectors like healthcare, where real-time image analysis can support rapid diagnosis and decision-making, and in autonomous systems, where low-latency image processing is essential for navigation and safety. Future advancements in hardware and algorithms will enable faster, more efficient image processing, bringing real-time capabilities to a broader range of applications.
3. Continuous Improvement in Accuracy and Generalization
The accuracy of image processing models will continue to improve as AI techniques evolve. Researchers are focusing on developing models that are better at generalizing, meaning they can handle a wider variety of images and tasks without overfitting. With advancements in transfer learning, unsupervised learning, and few-shot learning, models will become more adaptable and capable of learning from smaller, more diverse datasets. This will make them more effective across various domains, from medical imaging to industrial inspections, where the diversity of images can present challenges for traditional models.
4. Democratization of AI and Image Processing
In the future, we can expect a democratization of AI, with image processing models becoming more accessible to non-experts. Open-source frameworks and pre-trained models are already making it easier for developers and businesses to implement advanced image processing without requiring deep expertise in AI or machine learning. The trend towards low-code and no-code platforms will further simplify the integration of image processing models into applications, allowing smaller organizations or individual developers to harness the power of these models without extensive resources or technical knowledge.
5. Explainability and Ethical Considerations
As image processing models become more integrated into critical applications like healthcare, law enforcement, and finance, there will be a greater emphasis on explainability and transparency. The future of image processing models will likely see the development of more interpretable AI techniques, allowing users to understand how decisions are made. This will build trust in AI systems and ensure that they are used ethically. Moreover, regulations surrounding data privacy, bias, and fairness will become more stringent, pushing for responsible AI practices and reducing the potential for misuse in sensitive areas.
6. Fusion of Multi-Modal Data
The future of image processing will involve the integration of multiple data sources to provide richer insights. Combining image data with other types of data, such as audio, text, and sensor data, will create more powerful models capable of understanding complex situations. For instance, in medical diagnostics, models that combine imaging data with patient history, genetic information, and other biomarkers will allow for more accurate and comprehensive diagnoses. Multi-modal fusion will be particularly beneficial in industries like autonomous driving, where data from cameras, LiDAR sensors, and GPS need to be integrated to create a full understanding of the environment.
7. Automation of Image Annotation
As labeled data is crucial for training image processing models, automating the image annotation process will be a game-changer in the future. Advances in semi-supervised and unsupervised learning, as well as active learning, will allow models to label images automatically, reducing the need for manual intervention. This will significantly accelerate the development of high-quality datasets, making it easier to train models and apply them to a broader range of tasks. Automation will be especially beneficial in fields like medical imaging, where large, annotated datasets are critical for accurate diagnosis.
In short, the future of image processing models is marked by rapid advancements and exciting possibilities. With continued improvements in AI, edge computing, accuracy, and generalization, these models will become more accessible, efficient, and capable of handling increasingly complex tasks. As industries continue to adopt these technologies, image processing will play a crucial role in shaping the future of everything from healthcare and autonomous systems to entertainment and beyond.
Conclusion
In conclusion, new image processing models represent a significant leap forward in technology, offering transformative possibilities across various industries. From improved accuracy and real-time processing to the integration of AI and edge computing, these advancements are setting the stage for more efficient, accessible, and powerful image analysis capabilities. However, despite their immense potential, challenges such as high computational demands, data quality, and ethical considerations must be addressed to ensure their responsible and effective use.
As we look toward the future, the evolution of image processing models will continue to be shaped by breakthroughs in AI, machine learning, and hardware. The fusion of multi-modal data, improvements in explainability, and the increasing accessibility of these technologies will further expand their applications and impact. By overcoming current limitations and leveraging emerging technologies, the future of image processing holds great promise in revolutionizing industries like healthcare, security, entertainment, and beyond.
By embracing these innovations, businesses and professionals can unlock new opportunities, improve operational efficiency, and create more personalized and impactful experiences. The future of image processing models is undoubtedly bright, and their continued development will play a critical role in shaping the world around us.