Image-to-Video Synthesis Applications
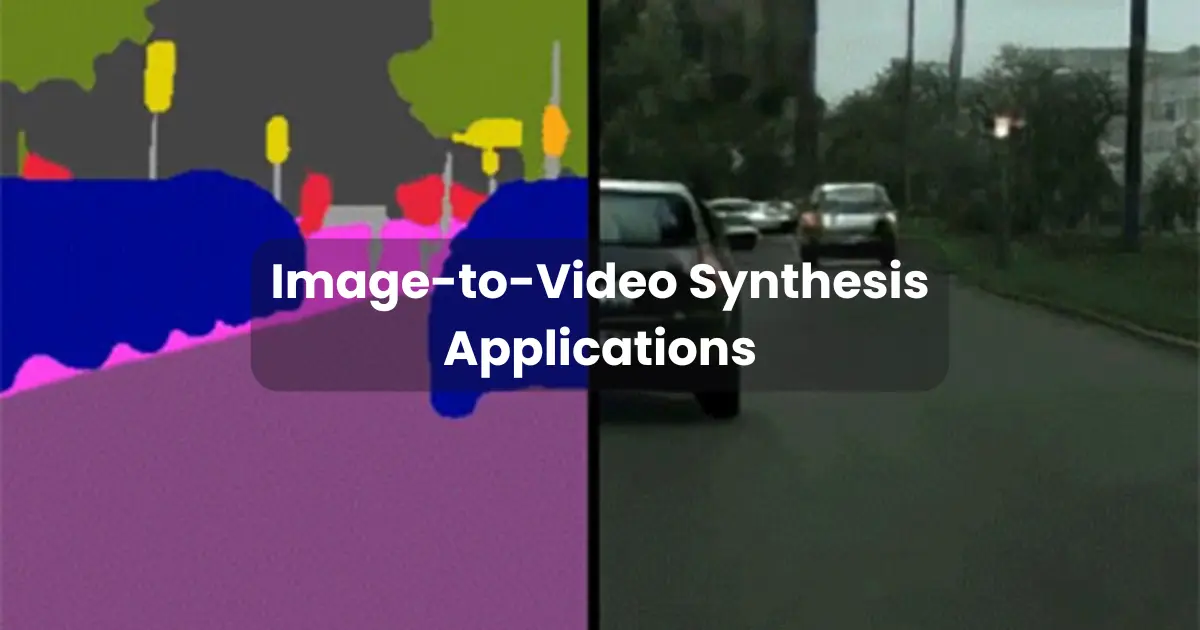
Contents
- 1 What is Image-to-Video Synthesis?
- 2 How Image-to-Video Synthesis Works
- 3 Applications of Image-to-Video Synthesis
- 3.1 1. Entertainment and Film Industry
- 3.2 2. Gaming and Virtual Reality (VR)
- 3.3 3. Social Media and Content Creation
- 3.4 4. Medical Imaging and Healthcare
- 3.5 5. Education and E-Learning
- 3.6 6. Digital Marketing and Advertising
- 3.7 7. Forensic Science and Security
- 3.8 8. Heritage Preservation and Deepfake Technology
- 3.9 9. Human-Computer Interaction and AI Avatars
- 4 Technologies Behind Image-to-Video Synthesis
- 4.1 1. Generative Adversarial Networks (GANs)
- 4.2 2. Recurrent Neural Networks (RNNs) and Long Short-Term Memory (LSTM) Models
- 4.3 3. Optical Flow Estimation
- 4.4 4. Neural Rendering and Style Transfer
- 4.5 5. 3D Pose Estimation and Motion Capture
- 4.6 6. Transformer-Based AI Models
- 4.7 7. Super-Resolution and Frame Interpolation
- 4.8 8. Cloud Computing and GPU Acceleration
- 5 Advantages of Image-to-Video Synthesis
- 5.1 1. Enhanced Content Creation
- 5.2 2. Cost and Time Efficiency
- 5.3 3. Realistic Motion Generation
- 5.4 4. Improved Accessibility for Content Creators
- 5.5 5. Versatility Across Industries
- 5.6 6. AI-Powered Personalization
- 5.7 7. Reviving Historical and Cultural Artifacts
- 5.8 8. Seamless Integration with Augmented Reality (AR) and Virtual Reality (VR)
- 5.9 9. Scalability for Large-Scale Video Production
- 5.10 10. Reduction of Manual Labor in Animation
- 6 Challenges and Limitations of Image-to-Video Synthesis
- 6.1 1. Lack of Realistic Motion Accuracy
- 6.2 2. High Computational Requirements
- 6.3 3. Data Dependency and Training Limitations
- 6.4 4. Ethical Concerns and Deepfake Misuse
- 6.5 5. Temporal Consistency Issues
- 6.6 6. Limited Generalization Across Diverse Input Images
- 6.7 7. Legal and Copyright Issues
- 6.8 8. Difficulty in Controlling Motion Parameters
- 6.9 9. Risk of Overfitting in AI Models
- 6.10 10. Storage and Bandwidth Limitations
- 7 Future Trends in Image-to-Video Synthesis
- 7.1 1. Improved Realism Through Advanced AI Models
- 7.2 2. Real-Time Video Synthesis
- 7.3 3. Ethical AI and Deepfake Detection Mechanisms
- 7.4 4. Enhanced User Control Over Motion Generation
- 7.5 5. Integration with Augmented Reality (AR) and Virtual Reality (VR)
- 7.6 6. AI-Powered Personalization in Marketing and Entertainment
- 7.7 7. Expansion in Healthcare and Medical Training
- 7.8 8. Democratization of AI-Generated Video Content
- 7.9 9. AI-Generated 3D Animation and Motion Capture
- 7.10 10. Legal and Regulatory Developments
- 7.11 Conclusion
In the rapidly evolving world of artificial intelligence, image-to-video synthesis applications have gained significant attention. These technologies transform static images into dynamic videos, creating realistic animations and lifelike motion sequences. As deep learning models improve, the ability to generate high-quality video content from a single image has opened up new possibilities in multiple industries. From entertainment and gaming to medical imaging and education, image-to-video synthesis applications are revolutionizing content creation and automation.
This article explores how image-to-video synthesis applications work, their key use cases, and the industries benefiting from this cutting-edge technology.
What is Image-to-Video Synthesis?
Image-to-video synthesis is an advanced artificial intelligence (AI) technique that generates dynamic video sequences from a single static image. By leveraging deep learning models, particularly generative adversarial networks (GANs) and neural rendering techniques, this process predicts motion and fills in missing frames to create realistic animations.
This technology enables AI systems to analyze an image, understand spatial structures, and simulate movement based on learned patterns. With improvements in machine learning, image-to-video synthesis applications have become more sophisticated, producing seamless transitions and high-quality video content.
As AI continues to evolve, image-to-video synthesis applications are transforming various industries by automating animation, enhancing user experiences, and generating lifelike digital content with minimal human intervention.
How Image-to-Video Synthesis Works
The process of image-to-video synthesis involves multiple AI-driven techniques that analyze a static image and generate a continuous video sequence. This transformation relies on deep learning models, motion prediction algorithms, and neural rendering methods. Below is a breakdown of the key steps in image-to-video synthesis applications:
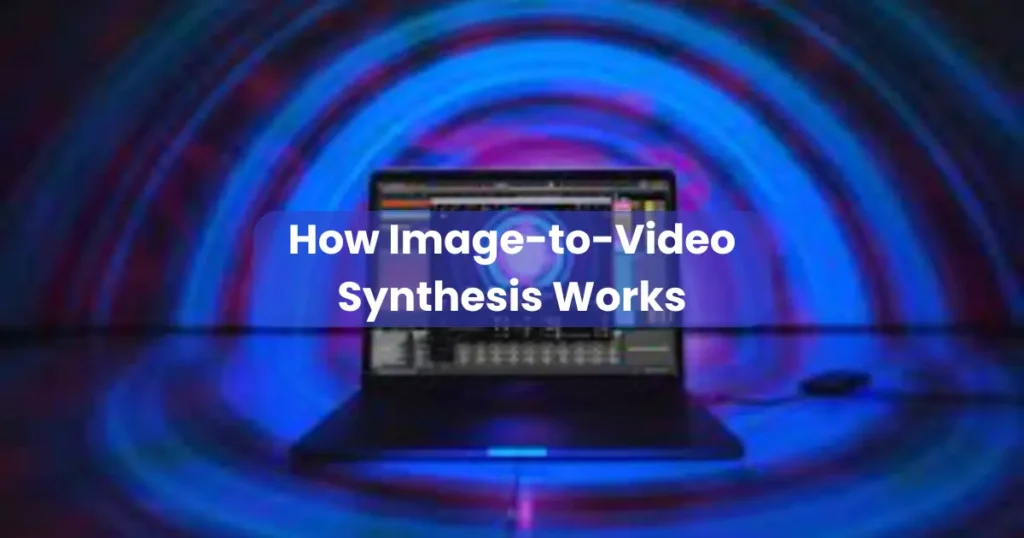
1. Image Analysis and Feature Extraction
Before generating motion, AI models extract crucial visual features from the input image. Convolutional Neural Networks (CNNs) detect edges, textures, and spatial structures to understand the image’s depth and composition.
2. Motion Prediction and Frame Generation
Once features are extracted, the system predicts possible motion based on training data. Using Recurrent Neural Networks (RNNs) and Generative Adversarial Networks (GANs), the AI estimates how objects in the image would move over time. Additional frames are generated by interpolating motion, ensuring smooth transitions between each stage.
3. Temporal Consistency Optimization
To maintain realism, AI models refine the generated frames, correcting inconsistencies such as unnatural distortions or abrupt motion changes. Techniques like optical flow estimation help track object movement, ensuring that the generated video looks seamless and coherent.
4. Post-Processing and Enhancement
After synthesis, AI-based upscaling techniques improve video resolution and color accuracy. Advanced tools like super-resolution models and frame interpolation refine details, making the final output more visually appealing and realistic.
With rapid advancements in AI, image-to-video synthesis applications continue to improve, enabling the creation of highly detailed, dynamic videos from single images with minimal computational effort.
Applications of Image-to-Video Synthesis
Image-to-video synthesis applications have transformed multiple industries by enabling the creation of realistic and dynamic video content from static images. With AI-driven advancements, this technology is being used for animation, education, healthcare, and various other fields. Below are some of the most significant applications of image-to-video synthesis:
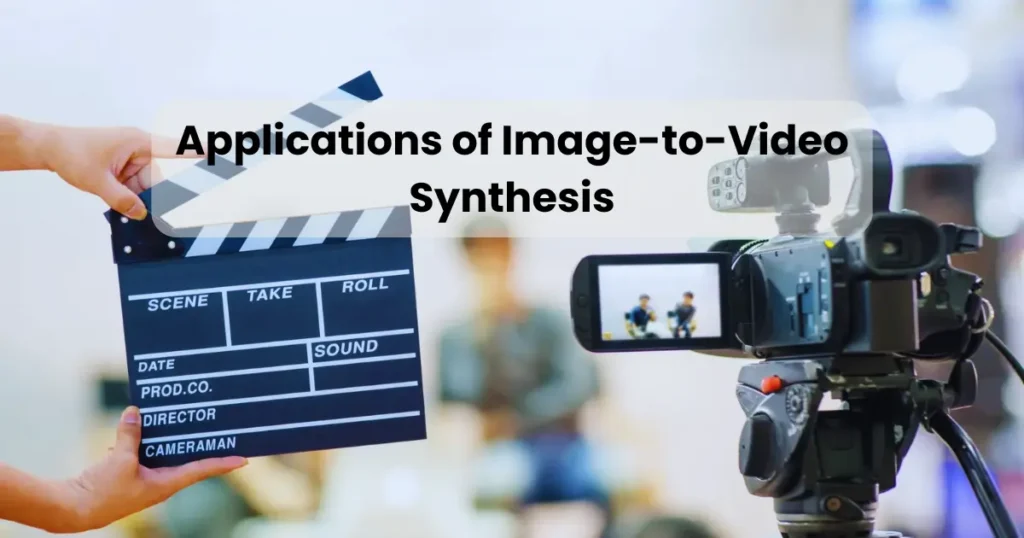
1. Entertainment and Film Industry
The entertainment sector has embraced image-to-video synthesis applications to enhance visual storytelling. Filmmakers use AI to animate historical figures, bring concept art to life, and generate realistic digital actors. This technology also plays a crucial role in visual effects (VFX), reducing production costs and streamlining animation workflows.
2. Gaming and Virtual Reality (VR)
Game developers use image-to-video synthesis to create dynamic character animations, lifelike facial expressions, and immersive VR environments. AI-powered motion synthesis allows for realistic character interactions, improving the overall gaming experience.
3. Social Media and Content Creation
Platforms like TikTok, Instagram, and YouTube leverage image-to-video synthesis applications to offer AI-driven animation tools. Users can animate photos, create personalized avatars, and generate engaging video content using minimal input. This has led to a surge in creative storytelling and interactive media.
4. Medical Imaging and Healthcare
In the healthcare sector, image-to-video synthesis applications assist in medical imaging by converting static scans into dynamic visualizations. AI-generated videos help doctors analyze patient conditions more effectively, simulate organ movements, and improve medical training by visualizing complex biological processes.
5. Education and E-Learning
Educational institutions use image-to-video synthesis to create interactive learning materials. Historical events, scientific concepts, and complex theories can be visualized dynamically, making learning more engaging. AI-generated instructional videos also help in language learning and skill development.
6. Digital Marketing and Advertising
Brands utilize image-to-video synthesis applications to produce eye-catching promotional content. AI-generated videos allow marketers to transform product images into dynamic advertisements, improving audience engagement and brand storytelling. Personalized video marketing has also become more accessible due to this technology.
7. Forensic Science and Security
Law enforcement agencies apply image-to-video synthesis for reconstructing crime scenes, enhancing surveillance footage, and predicting potential movements from still images. This helps in criminal investigations and security monitoring by providing more comprehensive visual data.
8. Heritage Preservation and Deepfake Technology
Museums and cultural institutions use image-to-video synthesis applications to restore historical artifacts, animate ancient paintings, and bring historical figures to life. However, the same technology also powers deepfakes, raising ethical concerns regarding misinformation and digital identity manipulation.
9. Human-Computer Interaction and AI Avatars
AI-powered virtual assistants and digital avatars benefit from image-to-video synthesis by enabling more natural human-computer interactions. Chatbots and virtual influencers use animated facial expressions and body language, enhancing user engagement and creating more lifelike AI personalities.
With continuous advancements in artificial intelligence, image-to-video synthesis applications are becoming more refined, efficient, and widespread across various fields. This technology is reshaping digital content creation and expanding the possibilities of AI-driven media.
Technologies Behind Image-to-Video Synthesis
The development of image-to-video synthesis applications relies on cutting-edge artificial intelligence (AI) models, deep learning techniques, and advanced computational frameworks. These technologies work together to transform static images into dynamic, high-quality video sequences. Below are the key technologies that power image-to-video synthesis:
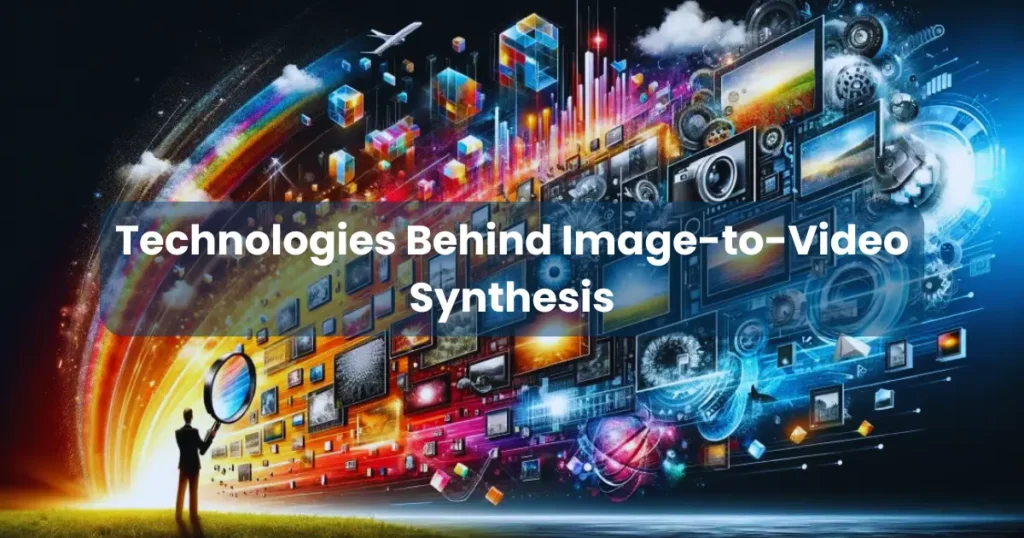
1. Generative Adversarial Networks (GANs)
GANs play a crucial role in image-to-video synthesis applications by generating realistic video frames from static images. A GAN consists of two neural networks:
- Generator – Creates new video frames by predicting motion and transformations.
- Discriminator – Evaluates the generated frames for authenticity and refines the output.
By continuously improving through competition, GANs produce high-quality, lifelike video sequences with smooth transitions.
2. Recurrent Neural Networks (RNNs) and Long Short-Term Memory (LSTM) Models
Since video synthesis requires temporal consistency, RNNs and LSTMs help maintain smooth motion by predicting frame sequences based on past data. These models enable image-to-video synthesis applications to generate realistic motion dynamics by analyzing spatial and temporal patterns.
3. Optical Flow Estimation
Optical flow estimation is used to track pixel movement between frames, ensuring that motion appears natural and continuous. This technique allows AI models to simulate object movement realistically, making image-to-video synthesis applications more accurate in motion prediction.
4. Neural Rendering and Style Transfer
Neural rendering techniques improve the quality of synthesized videos by refining textures, shadows, and lighting. Style transfer models help blend animations with artistic or cinematic effects, allowing users to apply different visual styles to AI-generated videos.
5. 3D Pose Estimation and Motion Capture
For applications requiring human motion synthesis, 3D pose estimation models analyze skeletal structures and predict natural movements. These models are widely used in image-to-video synthesis applications for animating digital avatars, deepfake technology, and virtual reality (VR) simulations.
6. Transformer-Based AI Models
Recent advancements in transformer-based architectures, such as Vision Transformers (ViTs), have improved the efficiency of image-to-video synthesis by enhancing contextual understanding. These models analyze image features with greater accuracy, resulting in smoother and more realistic animations.
7. Super-Resolution and Frame Interpolation
To enhance the visual quality of synthesized videos, AI-based super-resolution techniques upscale low-resolution frames while maintaining sharpness. Frame interpolation models generate additional in-between frames, ensuring smoother motion transitions in image-to-video synthesis applications.
8. Cloud Computing and GPU Acceleration
Real-time image-to-video synthesis requires high computational power. Cloud-based AI services and GPU acceleration optimize processing speed, enabling faster and more efficient video generation. Companies like NVIDIA, Google, and OpenAI are continuously improving AI hardware to support large-scale video synthesis.
With the rapid evolution of these technologies, image-to-video synthesis applications are becoming more advanced, offering higher realism, faster processing, and greater accessibility across various industries.
Advantages of Image-to-Video Synthesis
As artificial intelligence continues to evolve, image-to-video synthesis applications are offering numerous advantages across various industries. This technology is revolutionizing content creation by enhancing efficiency, reducing costs, and improving visual storytelling. Below are the key benefits of image-to-video synthesis:
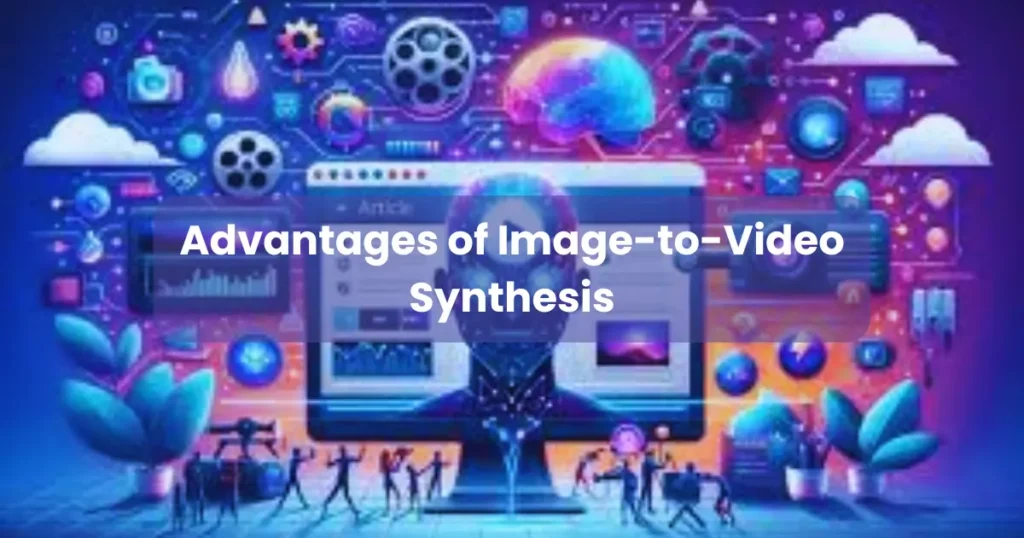
1. Enhanced Content Creation
One of the biggest advantages of image-to-video synthesis applications is their ability to generate high-quality video content from a single image. This enables artists, filmmakers, and marketers to create engaging visuals without extensive manual animation or video production efforts.
2. Cost and Time Efficiency
Traditional video production requires expensive equipment, professional actors, and significant time for editing. Image-to-video synthesis reduces these costs by automating animation and motion generation, allowing businesses to produce content faster and more affordably.
3. Realistic Motion Generation
With AI-powered motion synthesis, image-to-video synthesis applications create natural-looking animations that capture lifelike movement. These realistic animations are useful in fields such as gaming, virtual reality (VR), and character design.
4. Improved Accessibility for Content Creators
AI-driven image-to-video synthesis tools are making high-quality video production accessible to creators with limited technical skills. Social media users, digital marketers, and independent filmmakers can generate professional-looking content without specialized knowledge.
5. Versatility Across Industries
From medical imaging to forensic science, image-to-video synthesis applications are being used in diverse fields. Healthcare professionals use this technology for patient diagnosis, while law enforcement agencies leverage it for crime scene reconstruction and surveillance enhancement.
6. AI-Powered Personalization
Businesses can use image-to-video synthesis to create personalized content for their audiences. AI-generated animations can adapt to individual user preferences, allowing companies to enhance customer engagement with tailored video content.
7. Reviving Historical and Cultural Artifacts
Museums and historians utilize image-to-video synthesis applications to bring ancient figures and artworks to life. By animating historical portraits, this technology provides a more immersive way to explore history and culture.
8. Seamless Integration with Augmented Reality (AR) and Virtual Reality (VR)
As AR and VR technologies expand, image-to-video synthesis enhances immersive experiences by generating dynamic, AI-powered animations. Virtual environments and digital avatars benefit from AI-generated motion, creating more interactive and engaging applications.
9. Scalability for Large-Scale Video Production
AI-generated video synthesis enables mass production of animated content, making it ideal for marketing campaigns, educational programs, and automated news reporting. Image-to-video synthesis applications help scale content production while maintaining consistency and quality.
10. Reduction of Manual Labor in Animation
Traditional animation requires frame-by-frame drawing or motion capture. Image-to-video synthesis automates much of this process, reducing the need for manual intervention and speeding up animation workflows.
With continuous advancements in AI, image-to-video synthesis applications are becoming more refined, efficient, and widely adopted. This technology is reshaping how digital content is created, offering powerful solutions for multiple industries.
Challenges and Limitations of Image-to-Video Synthesis
Despite its numerous advantages, image-to-video synthesis applications face several challenges and limitations. While AI-powered video generation is improving rapidly, certain technical, ethical, and computational hurdles still need to be addressed. Below are the key challenges associated with image-to-video synthesis:
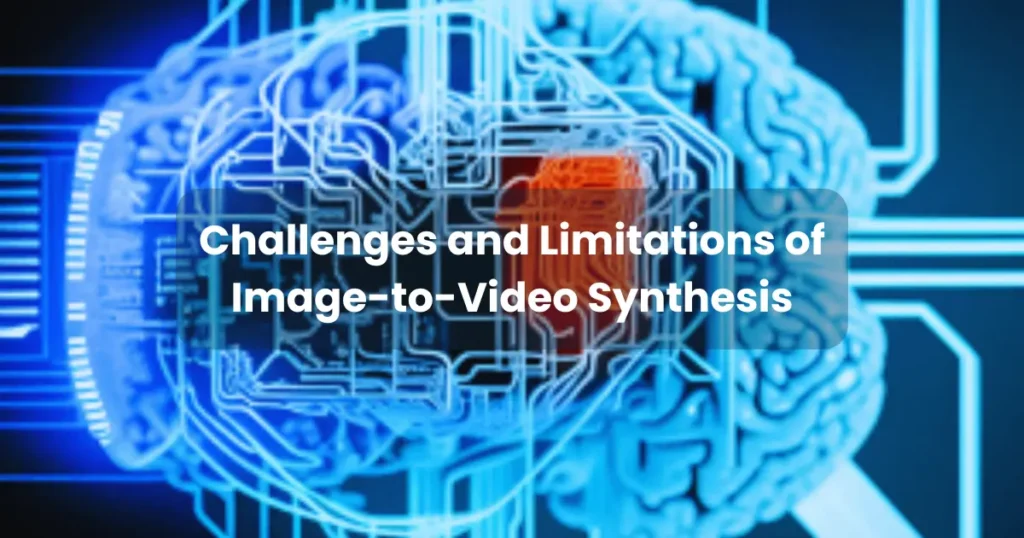
1. Lack of Realistic Motion Accuracy
One of the biggest challenges in image-to-video synthesis applications is generating truly realistic motion. AI models often struggle with natural movement, leading to unnatural distortions, jerky animations, or unrealistic transitions in generated videos.
2. High Computational Requirements
Deep learning models used for image-to-video synthesis require significant processing power, often relying on high-end GPUs and cloud computing resources. This makes real-time synthesis challenging for users without access to advanced hardware.
3. Data Dependency and Training Limitations
AI models need large datasets to generate high-quality videos. If the training data is biased or insufficient, image-to-video synthesis applications may produce low-quality or inaccurate animations. Additionally, training these models can be time-consuming and resource-intensive.
4. Ethical Concerns and Deepfake Misuse
While image-to-video synthesis has many positive applications, it also raises ethical concerns, particularly regarding deepfake technology. AI-generated videos can be used for misinformation, identity theft, and manipulative media, making it essential to implement strict regulations and detection mechanisms.
5. Temporal Consistency Issues
Ensuring smooth and consistent motion across generated frames remains a challenge. Some image-to-video synthesis applications struggle with maintaining temporal coherence, leading to flickering effects or sudden motion inconsistencies.
6. Limited Generalization Across Diverse Input Images
AI models may perform well on specific datasets but struggle when applied to diverse image types. Differences in lighting, resolution, or image quality can affect the accuracy of motion synthesis, making it difficult to achieve uniform results across all images.
7. Legal and Copyright Issues
The ability to generate videos from images raises legal questions regarding ownership and intellectual property rights. Unauthorized use of AI-generated videos could lead to copyright disputes, especially in industries such as media, marketing, and entertainment.
8. Difficulty in Controlling Motion Parameters
Users often face challenges in controlling specific motion patterns in image-to-video synthesis applications. While AI can predict movement, fine-tuning the speed, direction, or style of motion remains limited in many existing models.
9. Risk of Overfitting in AI Models
Some AI models may become too specialized in generating certain types of video sequences, leading to overfitting. As a result, the model may fail to generalize well when presented with new images outside of its training data.
10. Storage and Bandwidth Limitations
Generating high-resolution AI-driven videos requires large storage capacities and high-bandwidth processing. This can pose challenges for users who need to synthesize and distribute large amounts of AI-generated video content efficiently.
Despite these limitations, continuous advancements in AI and machine learning are helping to overcome many of these challenges. As image-to-video synthesis applications improve, they are expected to become more accessible, realistic, and ethically responsible in their usage.
Future Trends in Image-to-Video Synthesis
As artificial intelligence and deep learning continue to evolve, image-to-video synthesis applications are expected to become more sophisticated, accessible, and impactful across industries. Future advancements will focus on improving realism, efficiency, and ethical considerations. Below are some of the key trends shaping the future of image-to-video synthesis:
1. Improved Realism Through Advanced AI Models
Future AI-driven image-to-video synthesis applications will leverage more advanced neural networks, such as transformer-based models and diffusion models, to create ultra-realistic videos with highly accurate motion, lighting, and textures. These improvements will enhance the quality of AI-generated content in entertainment, gaming, and marketing.
2. Real-Time Video Synthesis
With the rise of powerful AI hardware and optimized algorithms, image-to-video synthesis is moving toward real-time processing. This will enable live applications, such as real-time facial animation for virtual influencers, AI-powered video conferencing avatars, and instant video editing for social media.
3. Ethical AI and Deepfake Detection Mechanisms
As image-to-video synthesis applications become more widespread, there is growing concern over their misuse, particularly in deepfake technology. Future developments will focus on creating AI-driven detection tools to identify and prevent fraudulent or deceptive AI-generated videos, ensuring ethical AI usage.
4. Enhanced User Control Over Motion Generation
One of the biggest limitations today is the lack of precise control over video output. Future image-to-video synthesis applications will offer advanced user interfaces that allow creators to define motion trajectories, adjust animation speed, and fine-tune specific details, making AI-generated videos more customizable.
5. Integration with Augmented Reality (AR) and Virtual Reality (VR)
With AR and VR technology gaining traction, AI-driven image-to-video synthesis will play a crucial role in creating immersive experiences. From generating lifelike virtual characters to animating historical figures in museums, AI-powered video synthesis will enhance how people interact with digital environments.
6. AI-Powered Personalization in Marketing and Entertainment
Brands and entertainment companies will leverage image-to-video synthesis applications to create personalized marketing campaigns, dynamic advertisements, and interactive storytelling. AI-generated videos tailored to individual preferences will enhance audience engagement and content consumption.
7. Expansion in Healthcare and Medical Training
Future applications of image-to-video synthesis in healthcare will include AI-generated medical simulations, animated patient records, and real-time procedural training. This technology will help medical professionals visualize complex conditions and improve diagnosis accuracy.
8. Democratization of AI-Generated Video Content
As AI technology becomes more accessible, image-to-video synthesis applications will no longer be limited to large corporations. Small businesses, independent creators, and educators will have access to powerful AI tools for generating video content without extensive technical knowledge.
9. AI-Generated 3D Animation and Motion Capture
Future advancements will enable AI to generate 3D animations from 2D images, reducing the need for traditional motion capture technology. This will revolutionize industries like film production, gaming, and virtual influencers, making animation more cost-effective and efficient.
10. Legal and Regulatory Developments
With the increasing adoption of AI-generated videos, governments and organizations will implement regulations to ensure responsible use. Legal frameworks will address copyright issues, ethical considerations, and the responsible deployment of image-to-video synthesis applications to prevent misuse.
Conclusion
The rapid advancements in image-to-video synthesis applications are transforming digital content creation, making it more accessible, efficient, and innovative. This AI-driven technology enables users to generate realistic video sequences from static images, revolutionizing industries such as entertainment, marketing, healthcare, and education.
Despite its numerous advantages, image-to-video synthesis still faces challenges, including motion inaccuracies, high computational requirements, and ethical concerns surrounding deepfake misuse. However, ongoing research and technological improvements are addressing these limitations, paving the way for more refined and responsible AI applications.
Looking ahead, the future of image-to-video synthesis is promising. With developments in real-time processing, AI-powered personalization, AR/VR integration, and enhanced motion control, this technology will continue to redefine how videos are created and consumed. As AI models become more sophisticated, businesses, content creators, and researchers will be able to leverage image-to-video synthesis applications to push the boundaries of storytelling and visual communication.
In conclusion, image-to-video synthesis is a groundbreaking innovation with limitless potential. By balancing technological progress with ethical considerations, this AI-driven revolution will shape the future of digital media, unlocking new creative possibilities across multiple domains.